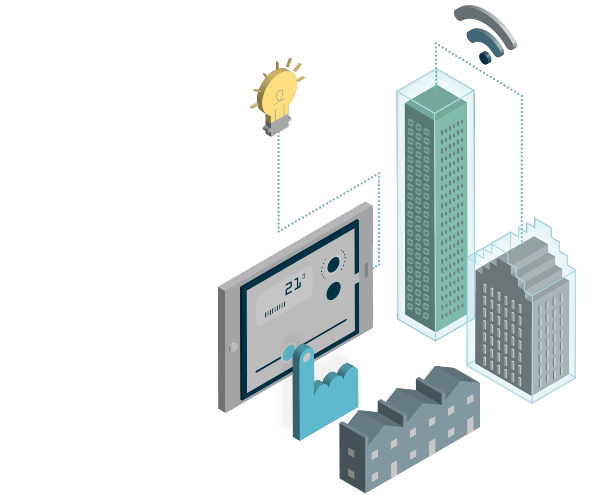
Smart cities are enhancing our way of life through improved places of work, better living and superior travel. But smart cities are also so much more than that.
The vision of a smart city requires interoperability of technology and standards, net zero energy consumption, new finance models and the move from single-asset-based thinking to interdependent city-wide assets built on a platform of connectivity and open data.
As established smart cities experts, we regularly work alongside our clients from across the finance, technology, infrastructure, energy, transport, healthcare and public sectors in thinking about, discussing and preparing for smart cities.
Our experience means that we're able to advise on some of the key issues that will inform the development of smart cities over the next few years. This includes data sharing, data interoperability, cyber security, finance, the public-private interface and, in particular the development of the framework of regulation and standards on which this interoperable digital infrastructure will be built.
If your business is facing the corporate and commercial, data, cyber security and regulatory challenges involved in creating smart city infrastructure, our experts can help.
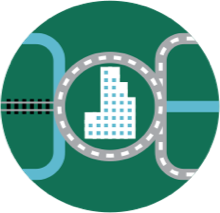
Case study | Chorus Life project
Find out about our work with Costim Group on the Chorus Life project, which relates to the development of a smart city district in Bergamo.
Connect with one of our experts
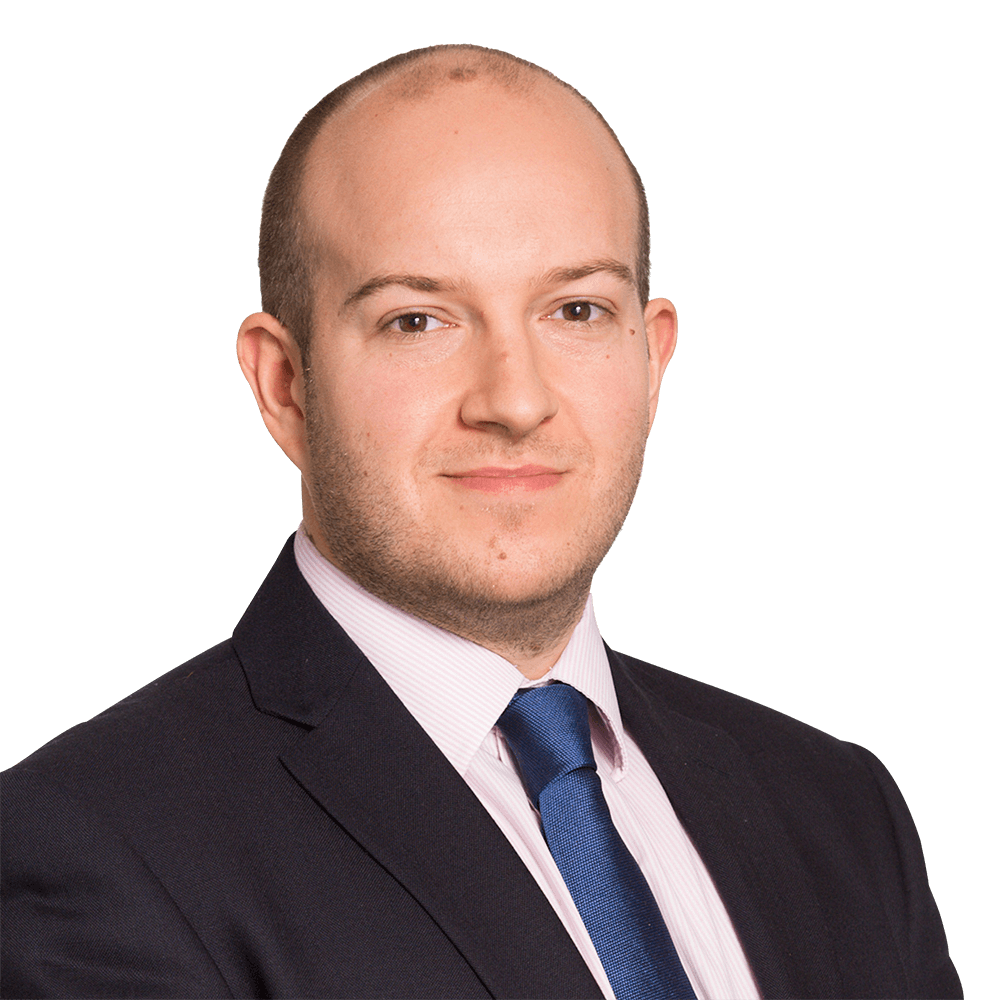
Jonathan Mills
Partner, UK
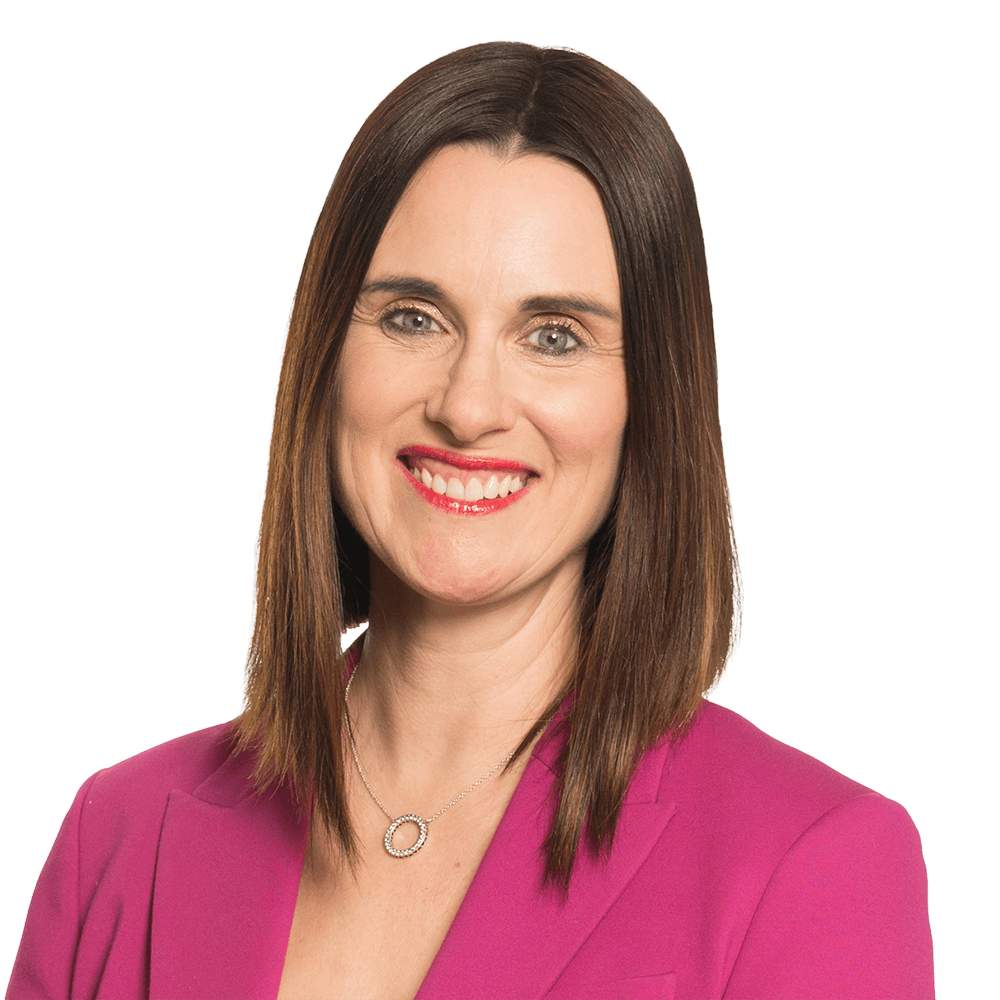
Olivia Sinfield
Partner, Head of Urban Dynamics, UK
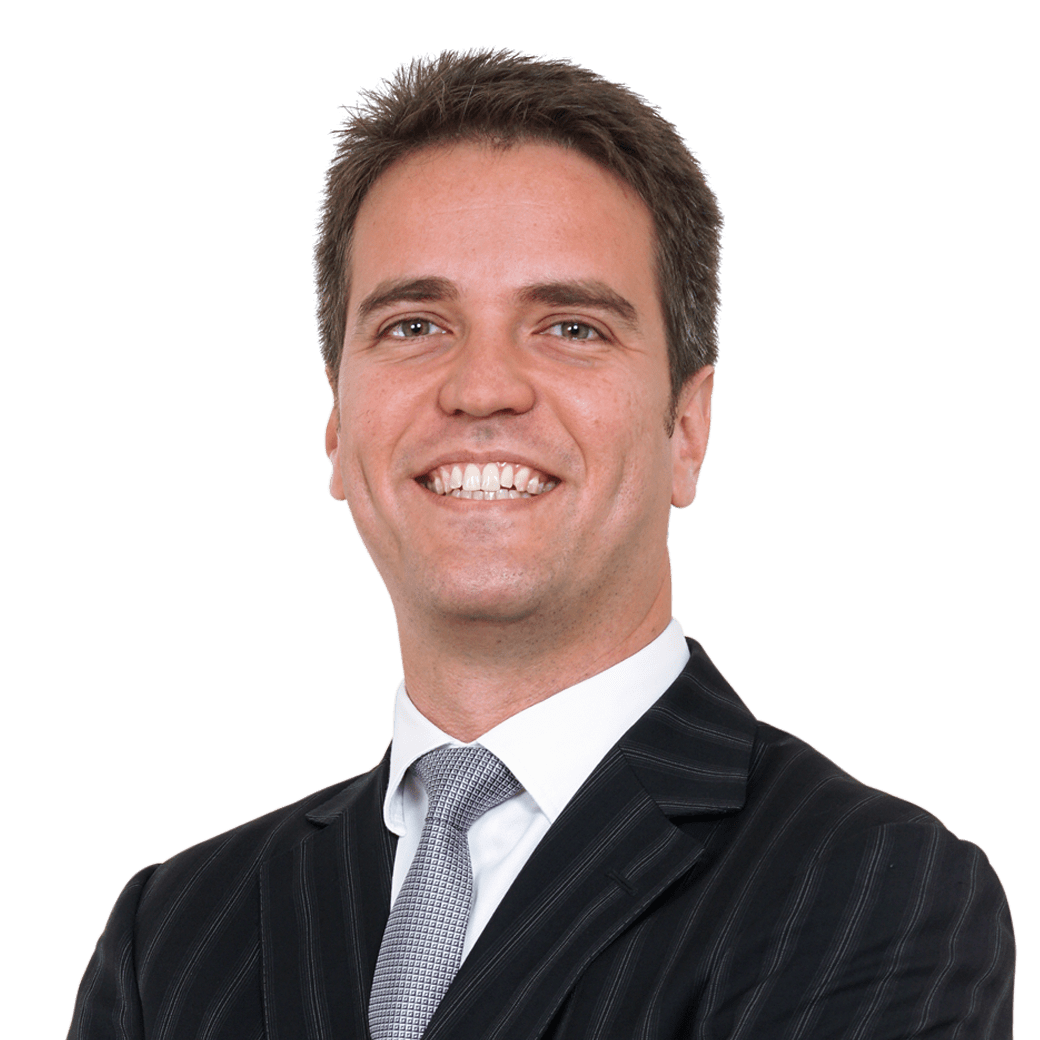
Julián Matos
Partner, Spain
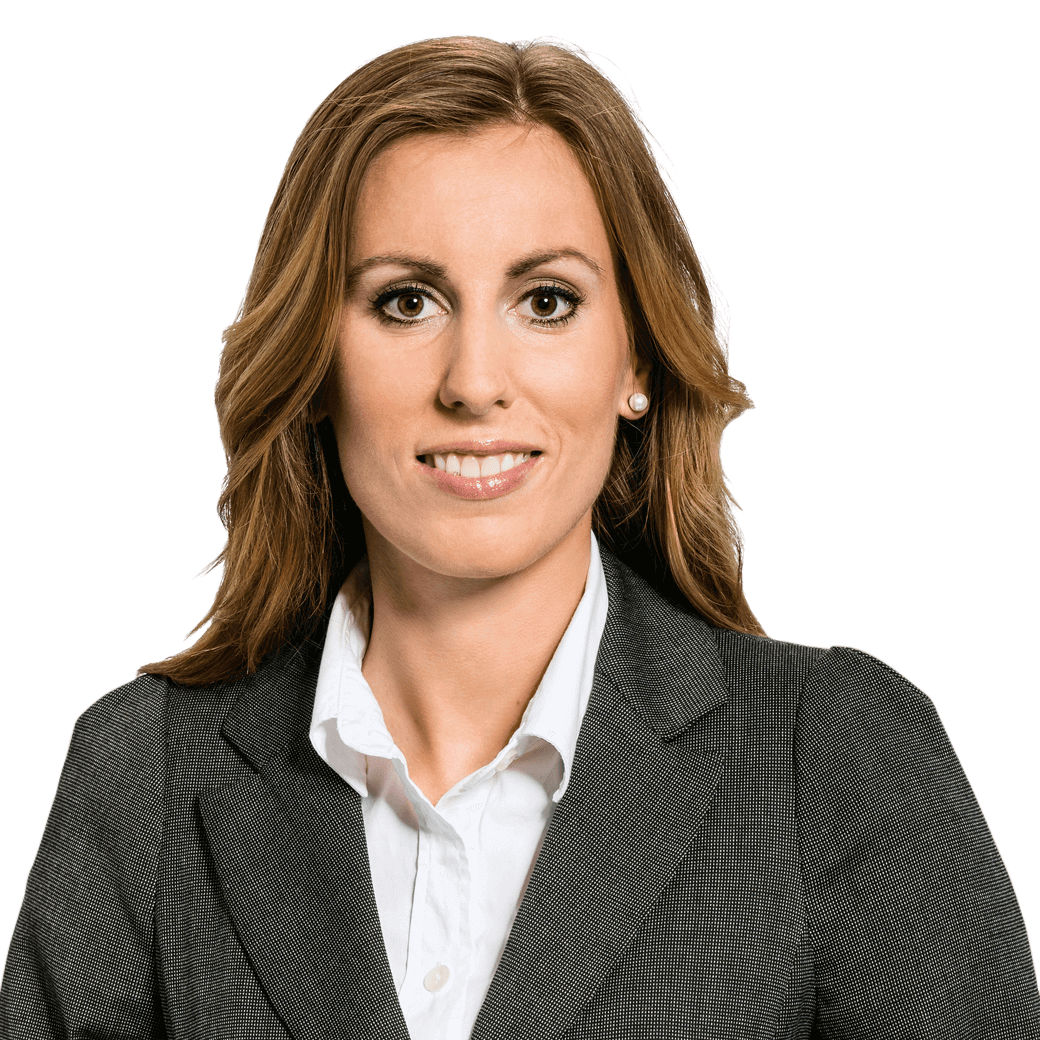
Dr Viktoria Winstel
Partner, Specialist lawyer for employment law, Germany
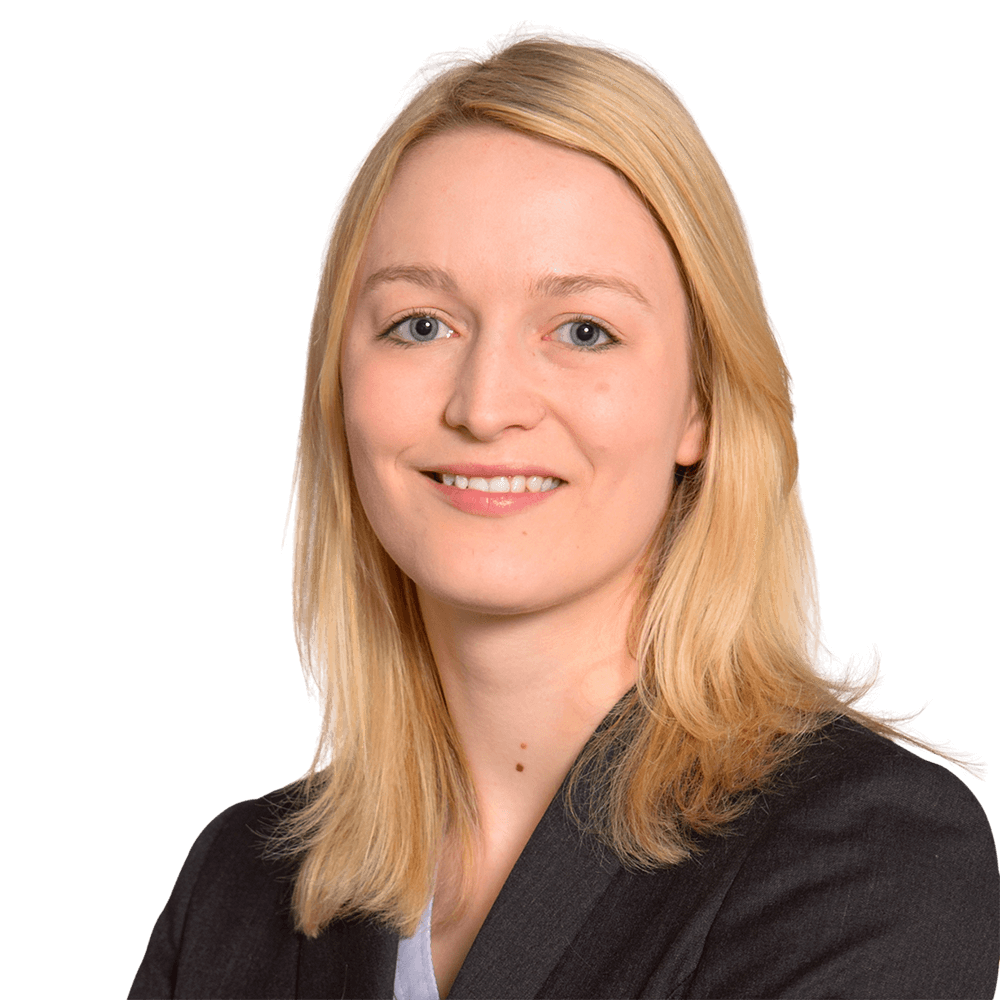
Becky Crawford
Associate Director, UK
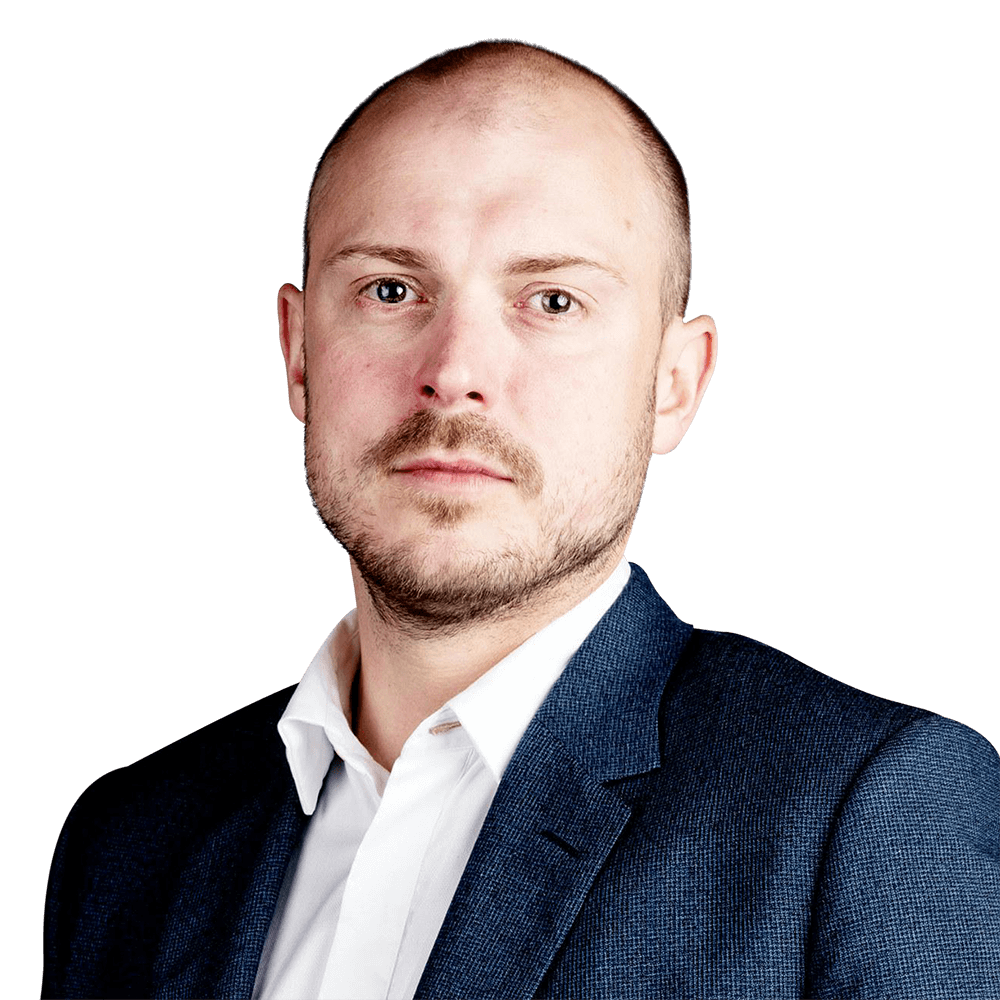
Tom Andrews
Associate Director, Transformation Manager - Urban Dynamics, UK
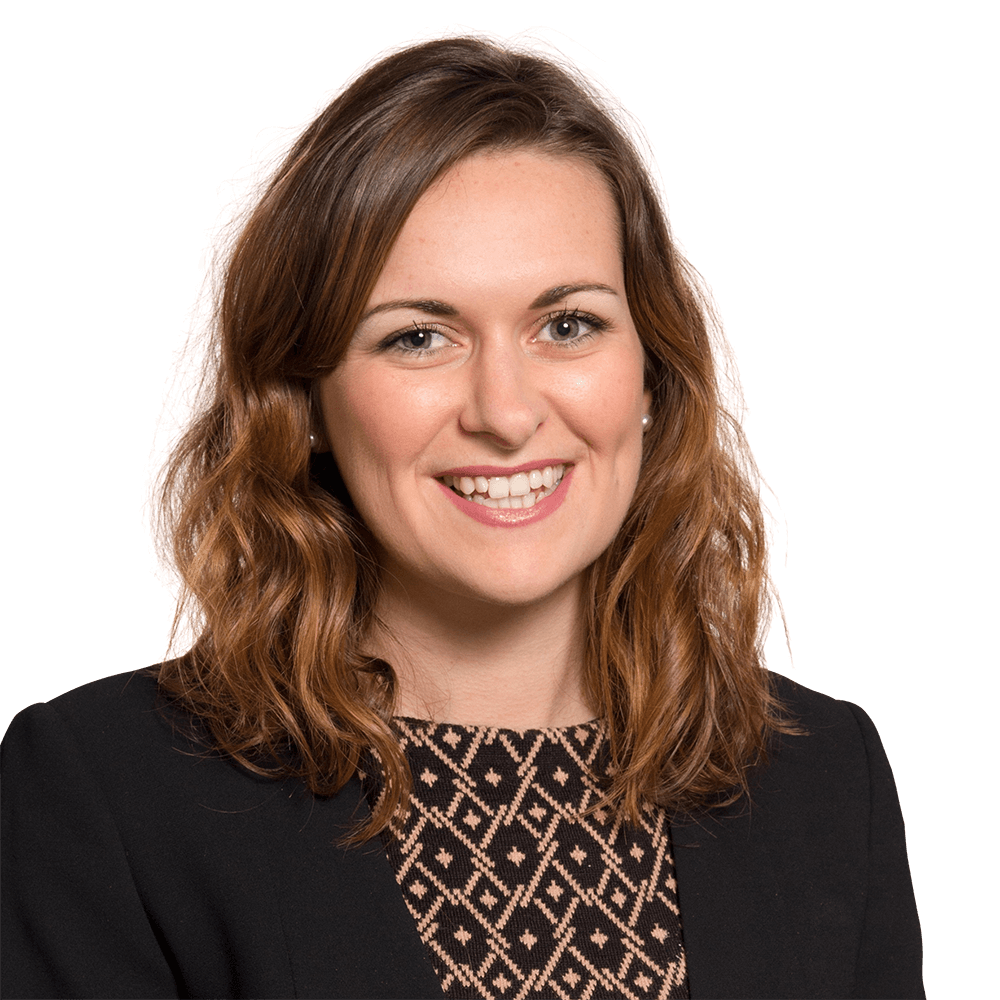
Elinor Owen
Associate Director, UK
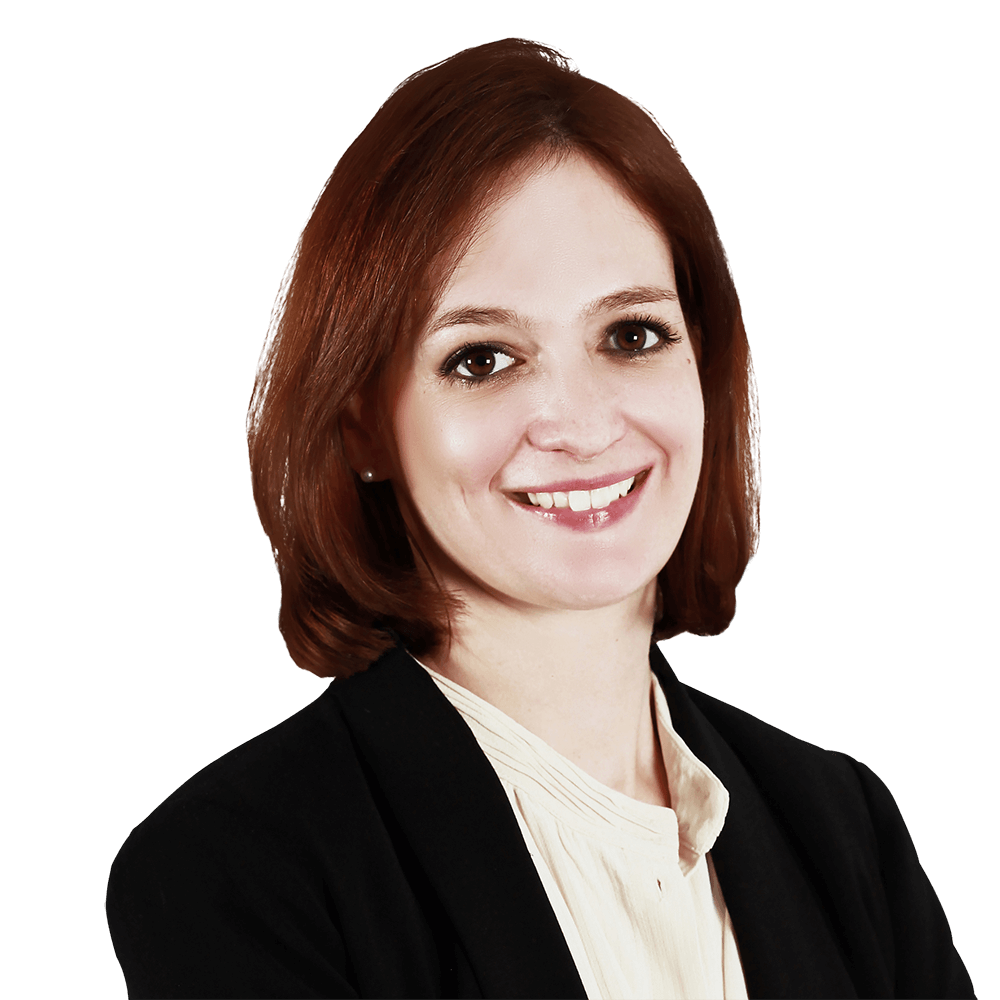
Emmanuelle Yvon
Counsel, France
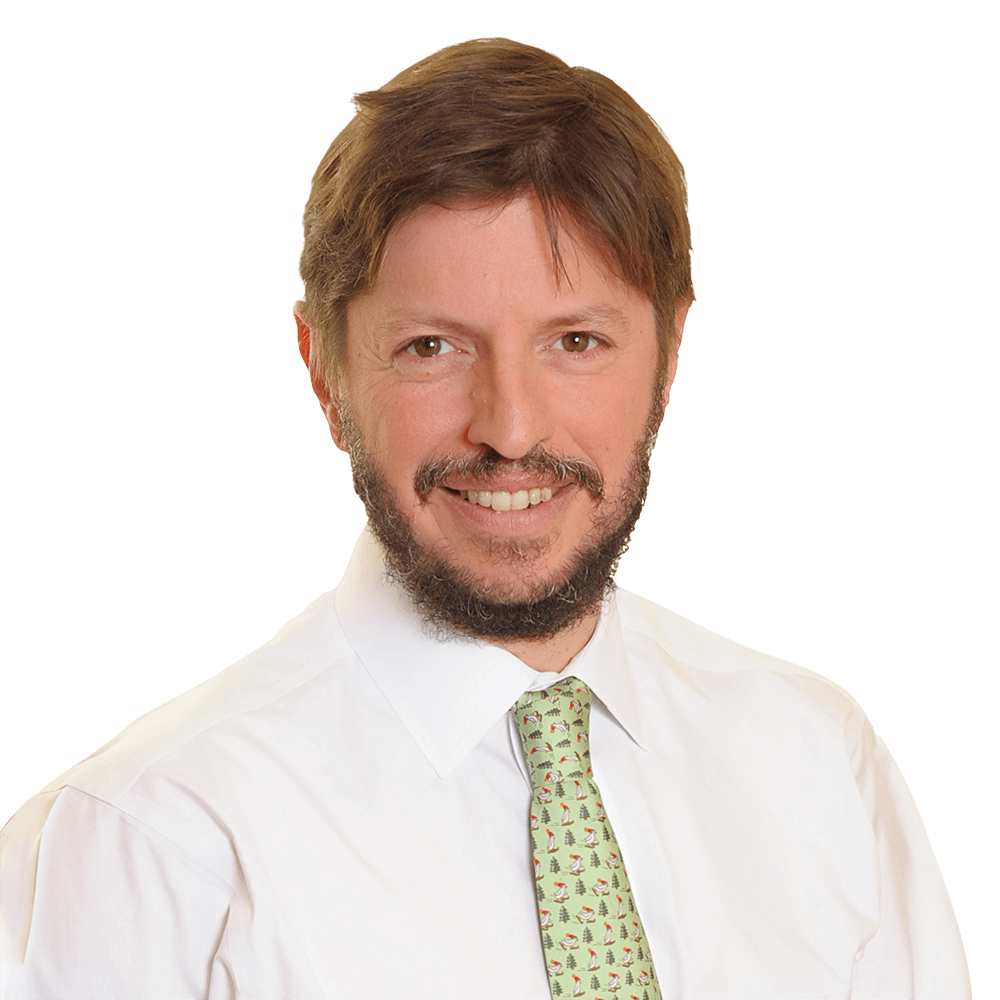
Simone Monesi
Partner, Head of Urban Dynamics, Italy
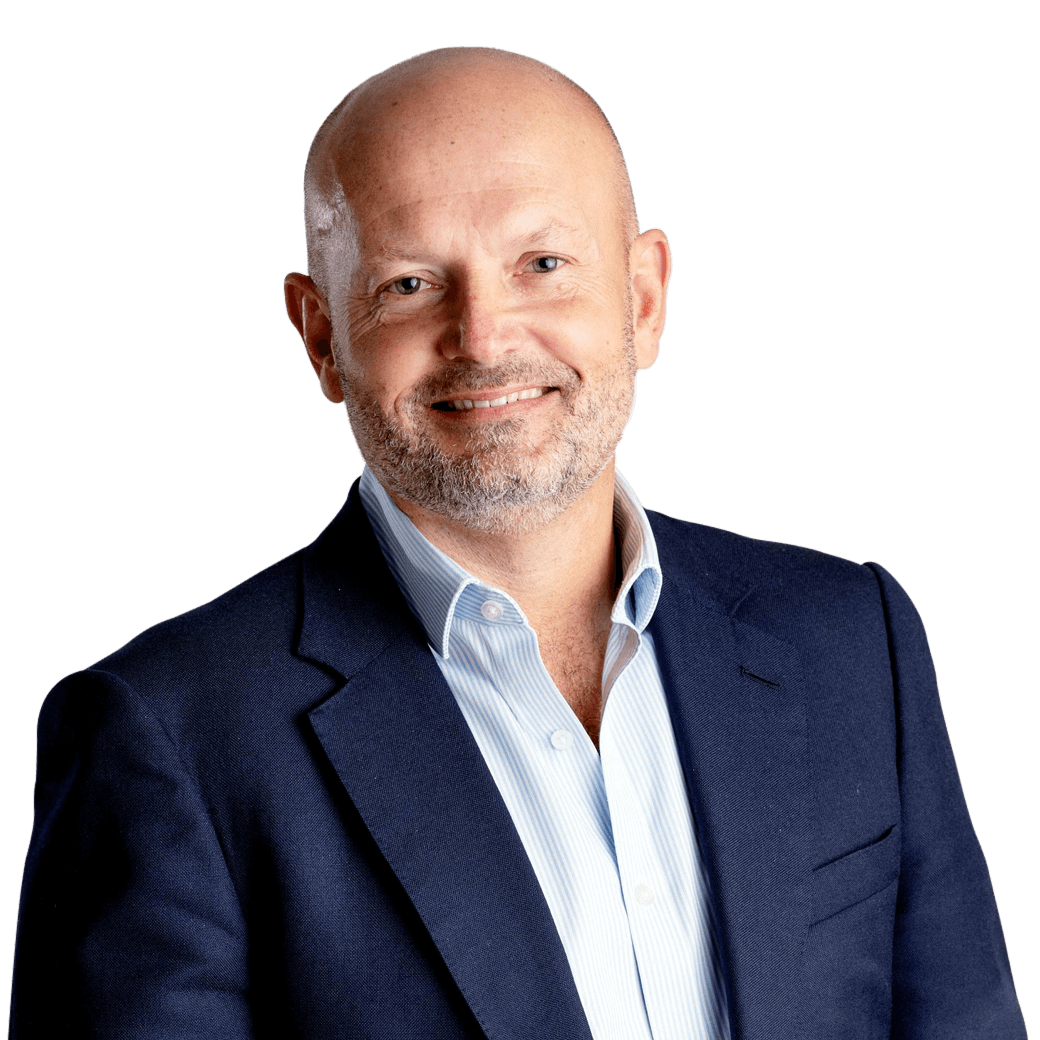
Conrad Davies
Managing Partner, UK
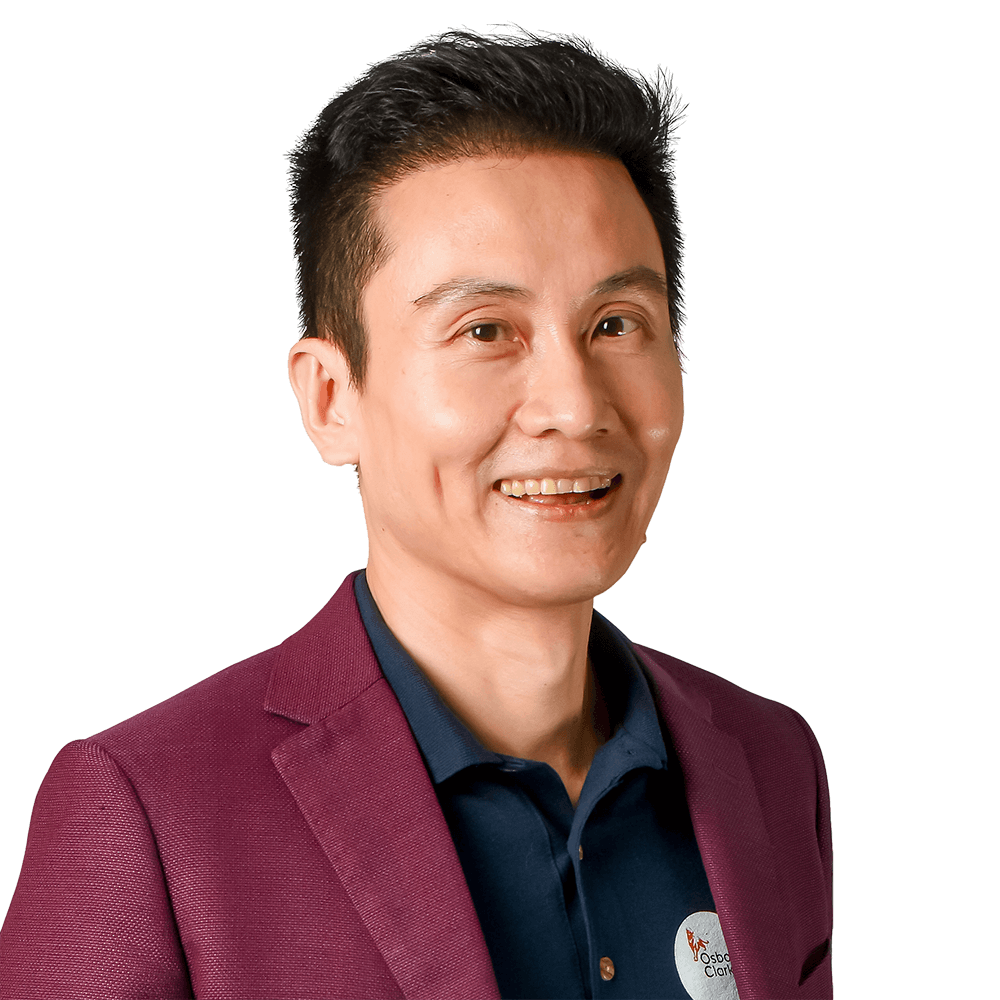
Chia-Ling Koh
Managing Director, Singapore
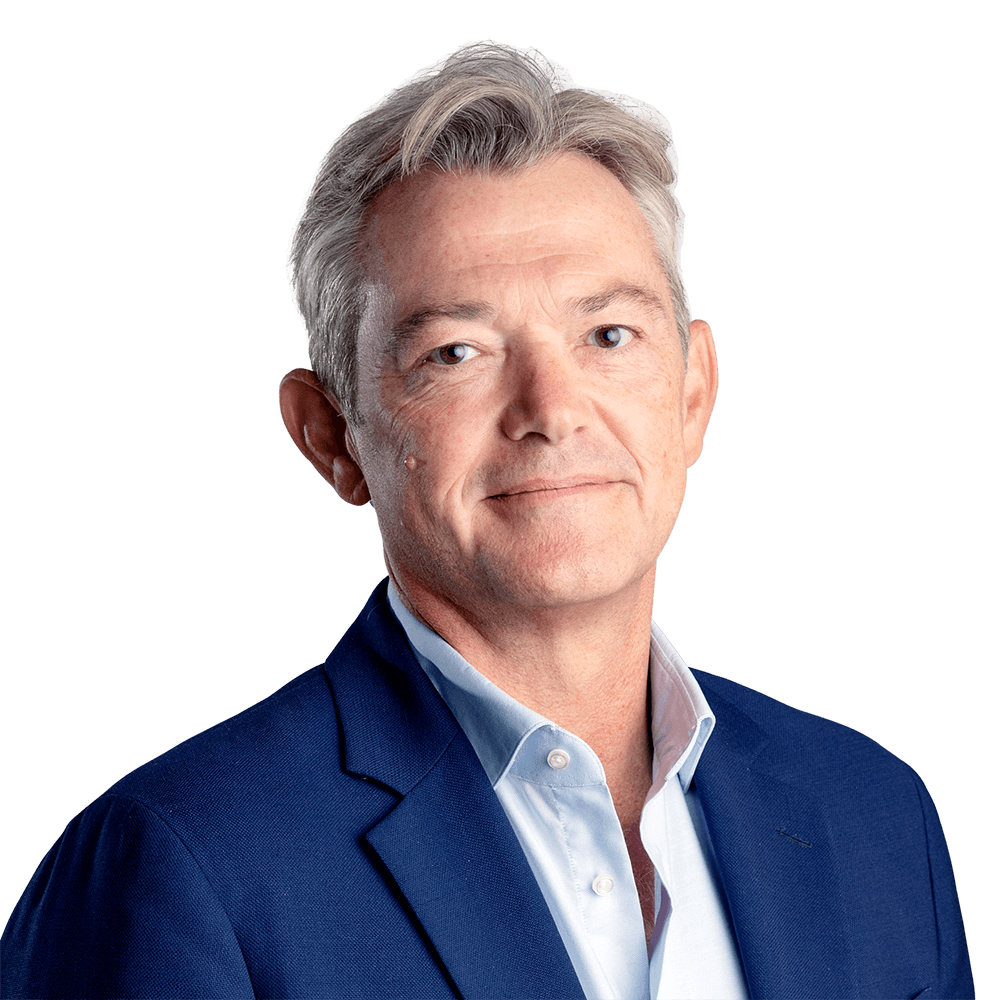
Simon Spooner
Partner, Head of Urban Mobility, UK
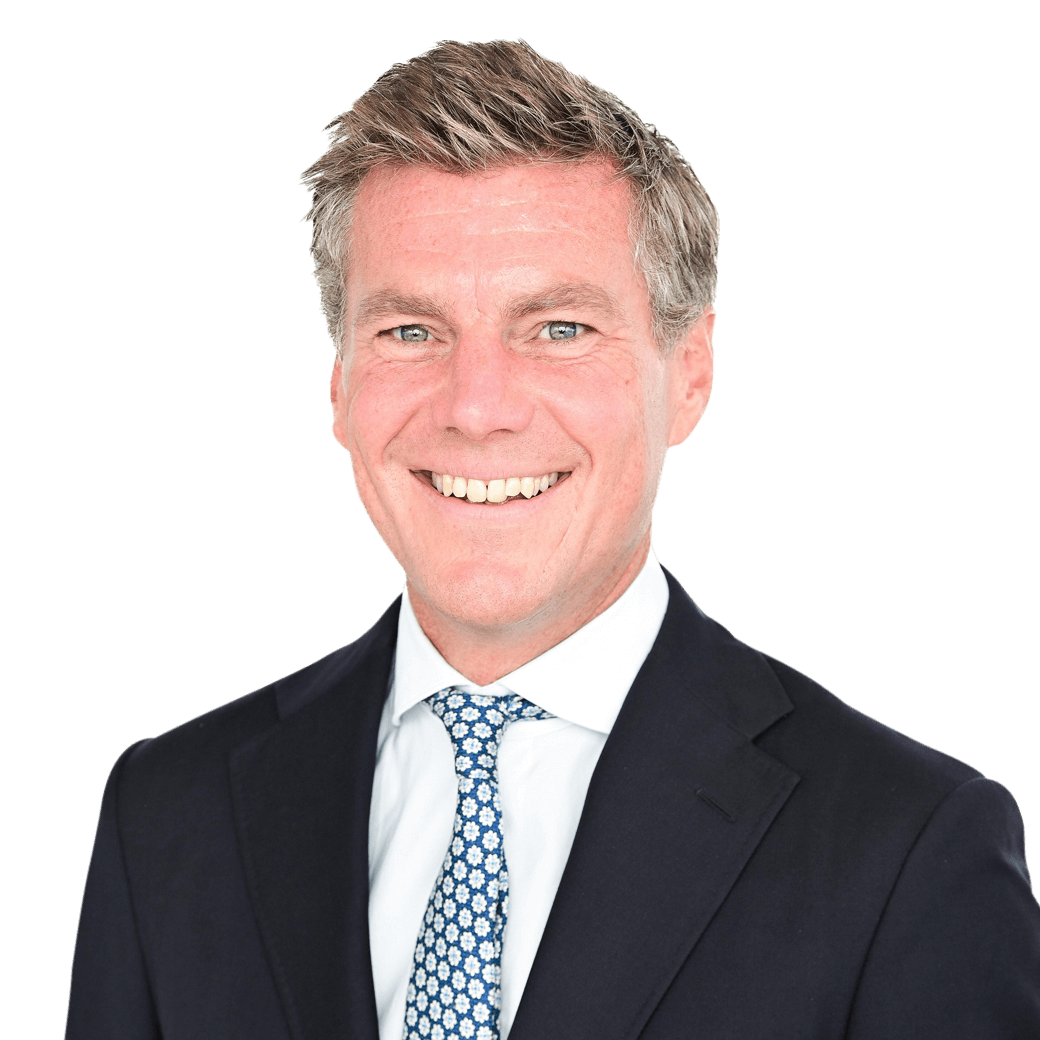
Berend Zwart
Partner, Lawyer, The Netherlands
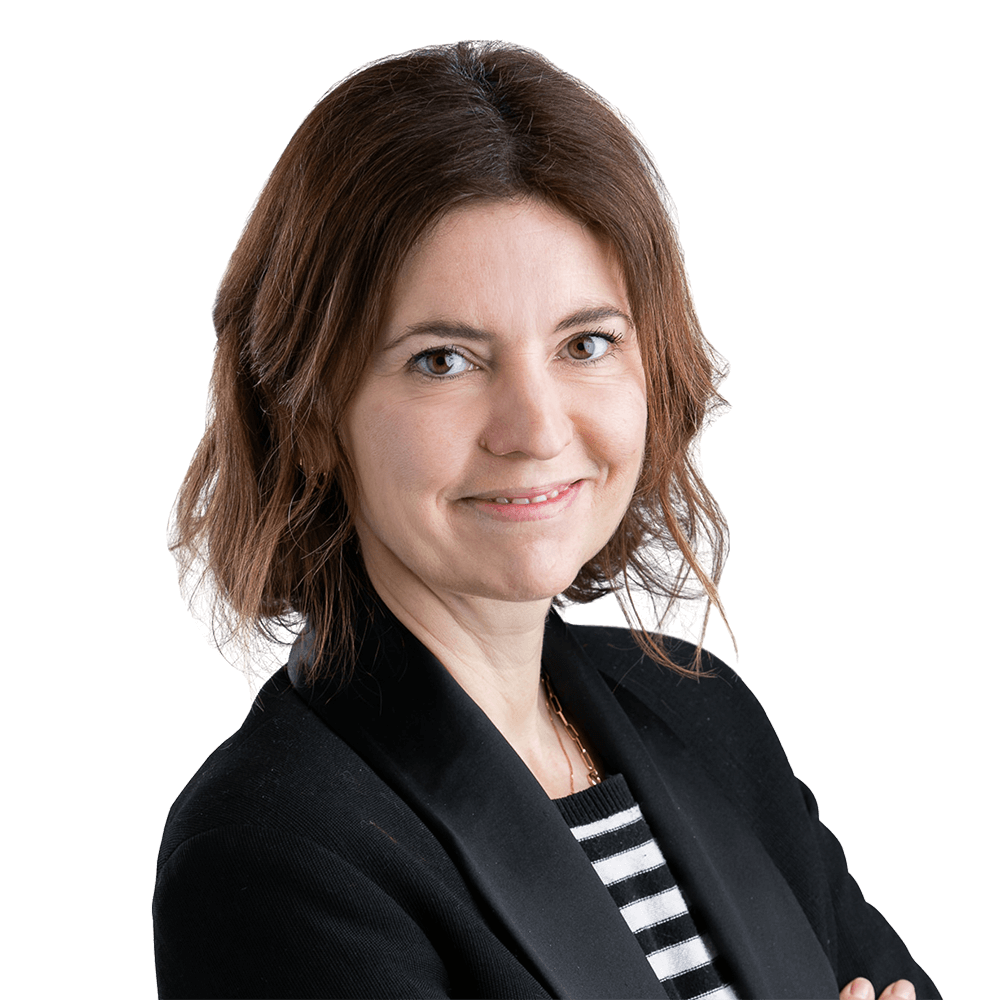
Vinciane Rysselinck
Senior Counsel, Belgium
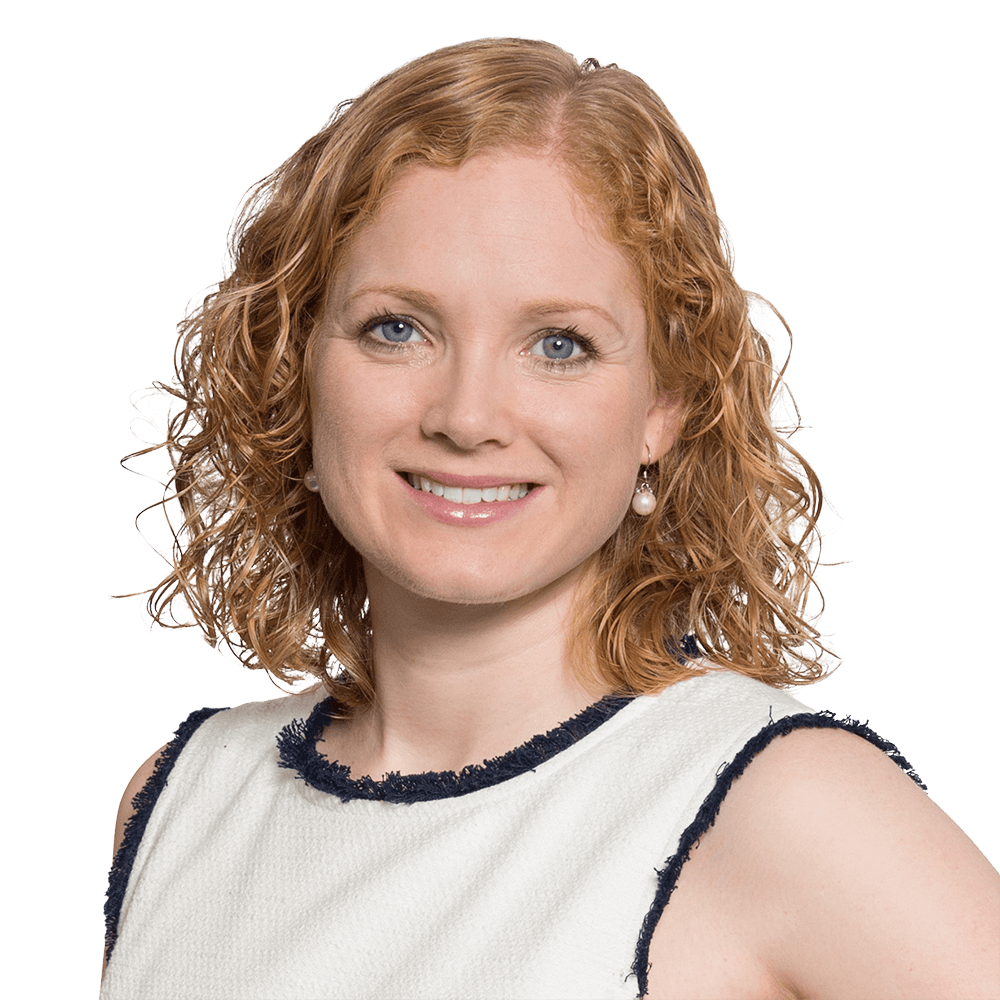
Eleanor Williams
Partner, UK
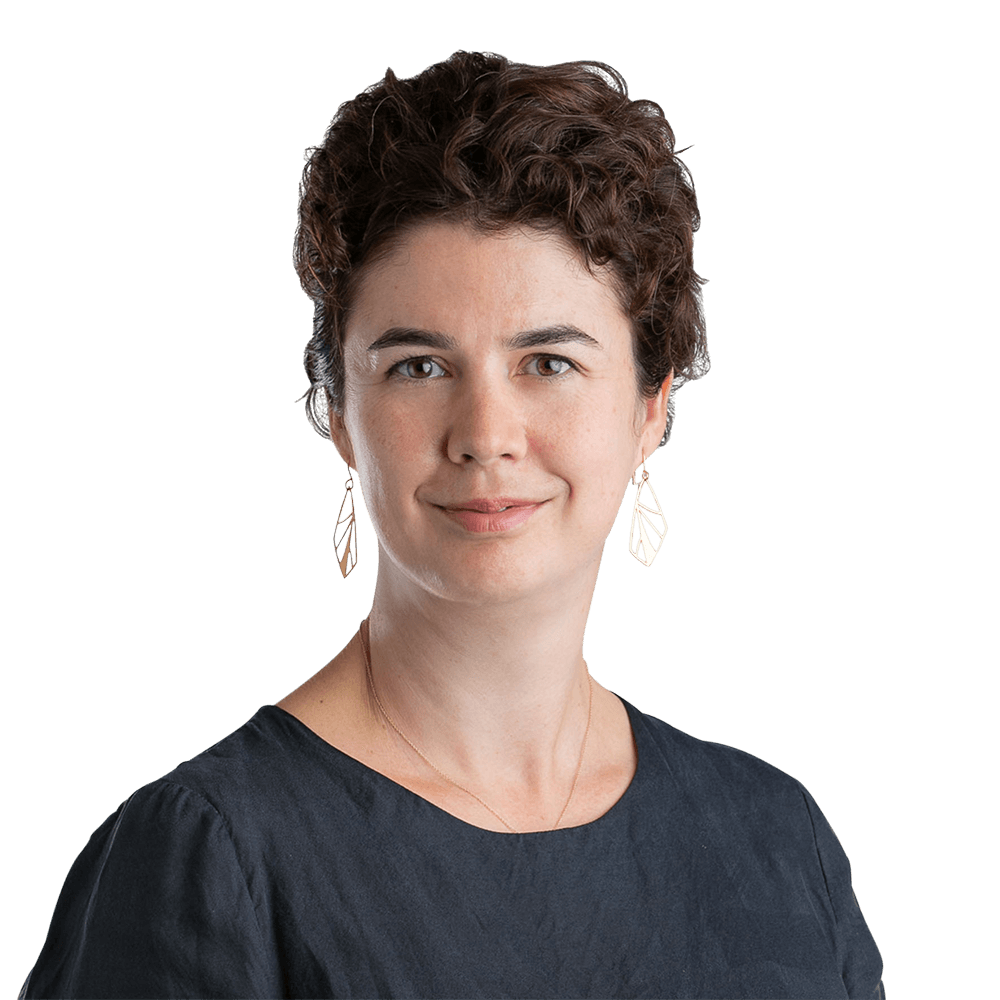
Fran Claes
Partner, Head of The Built Environment, Belgium
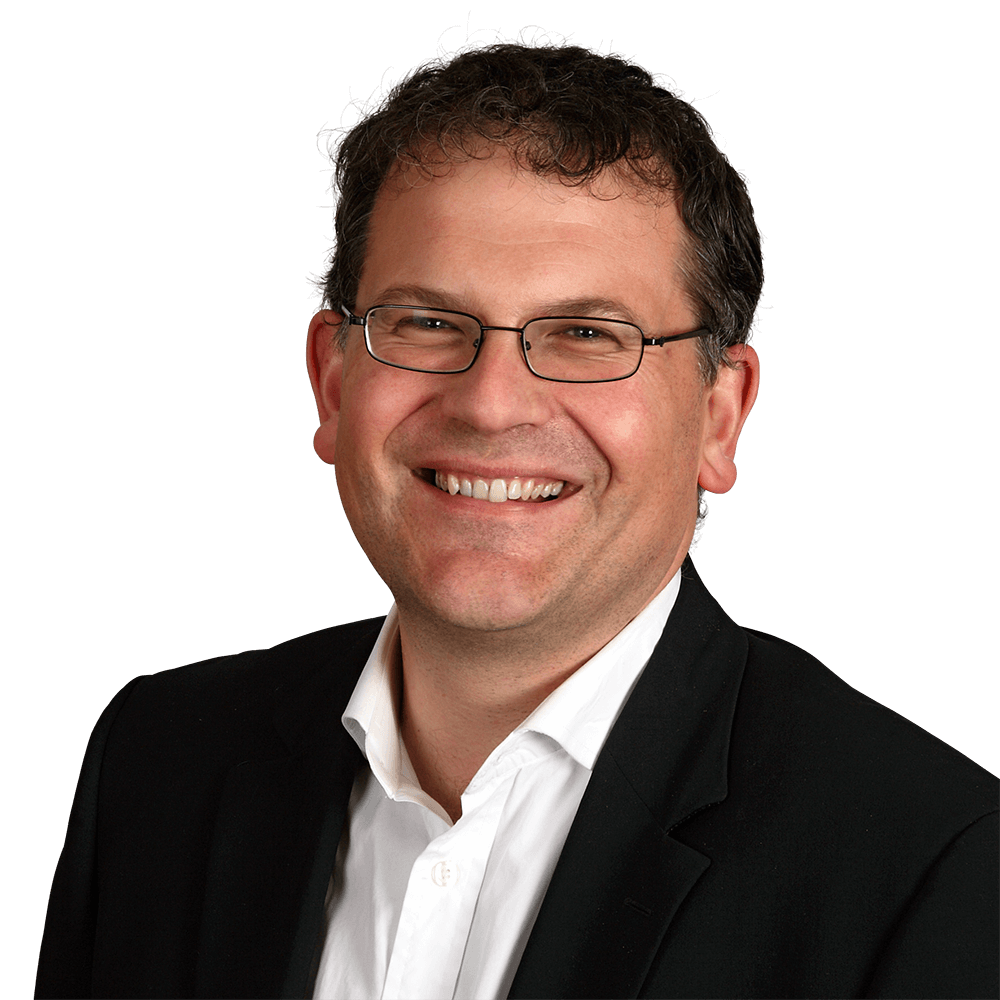
John Baird
Partner, UK
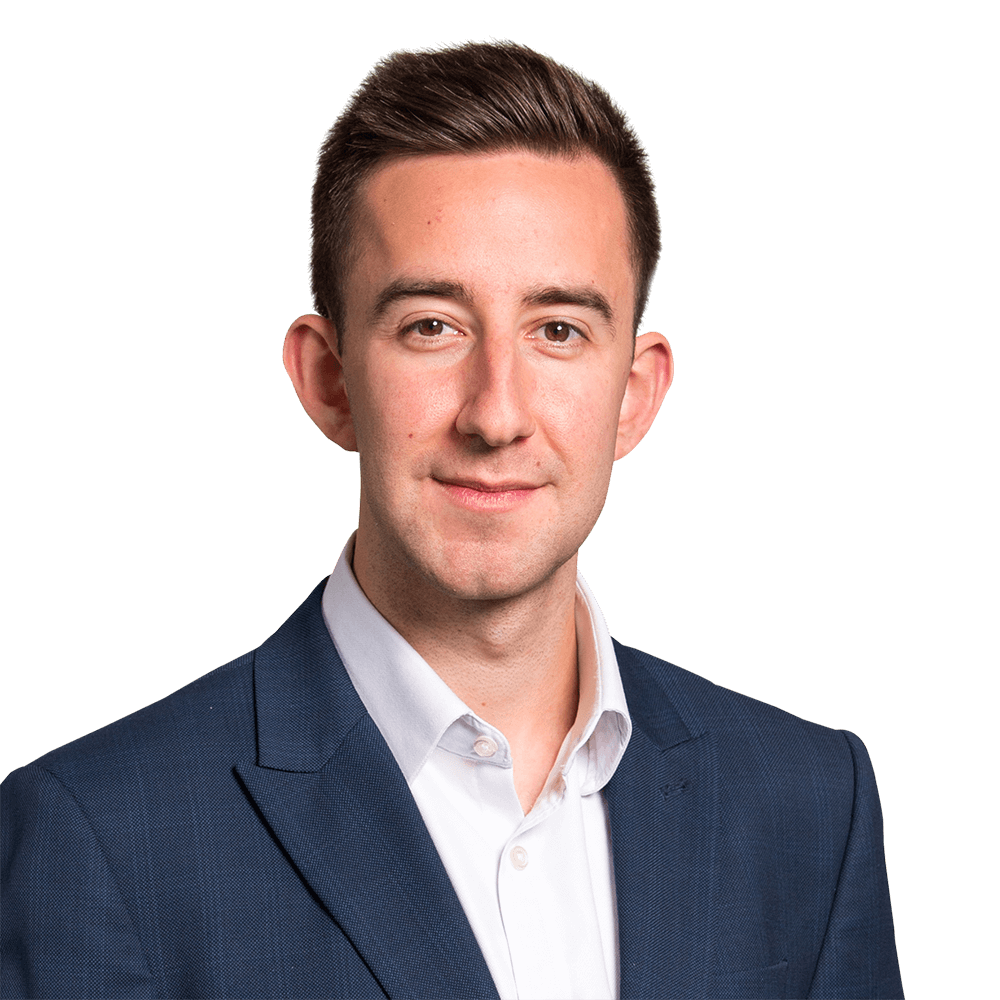
Daniel Stewart
Senior Associate, UK
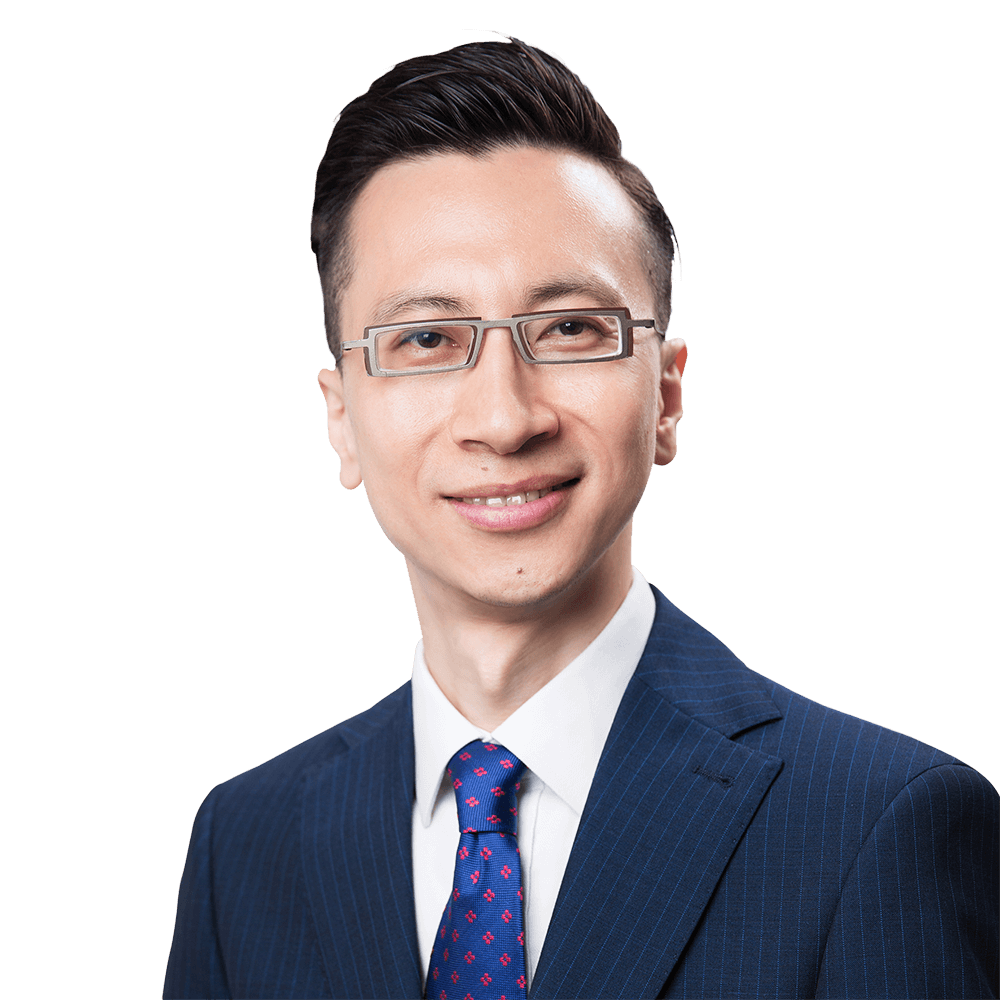
Steve Yu
Managing Partner, Co-founder, China
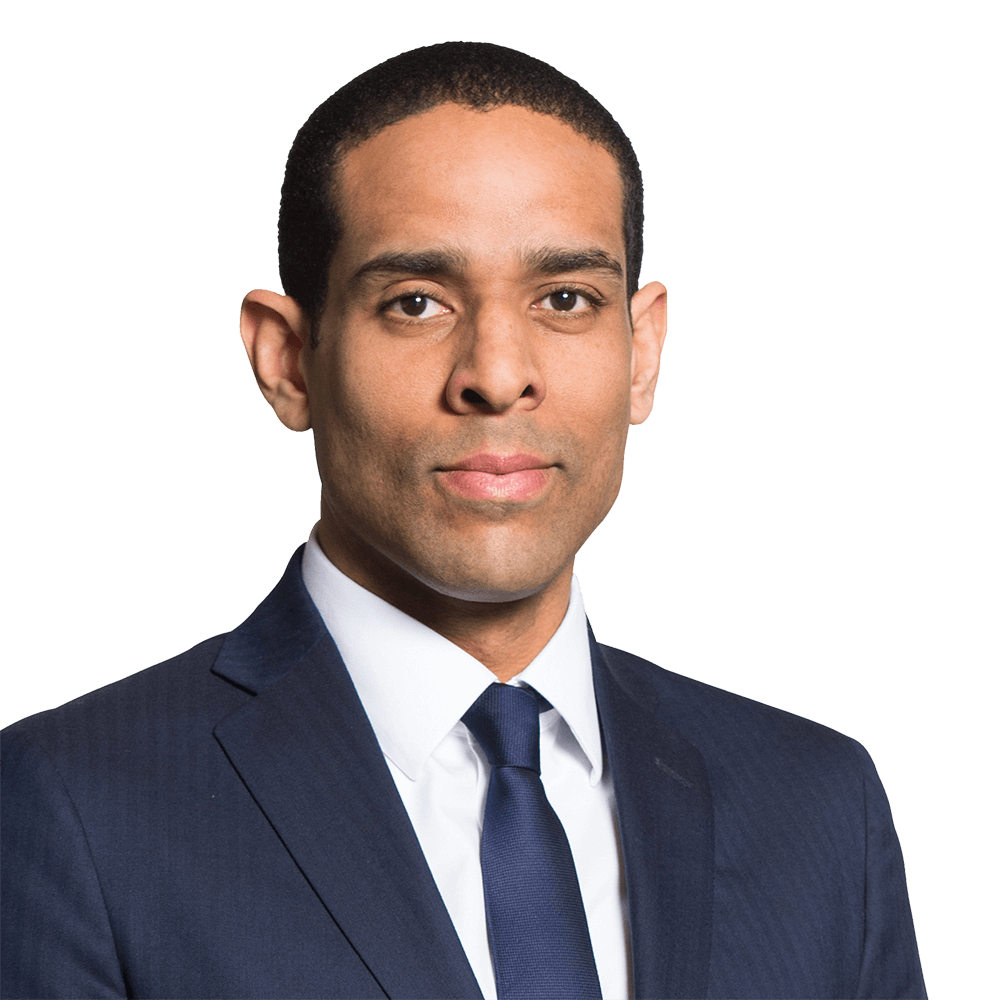
Robert Adjetey
Partner, UK
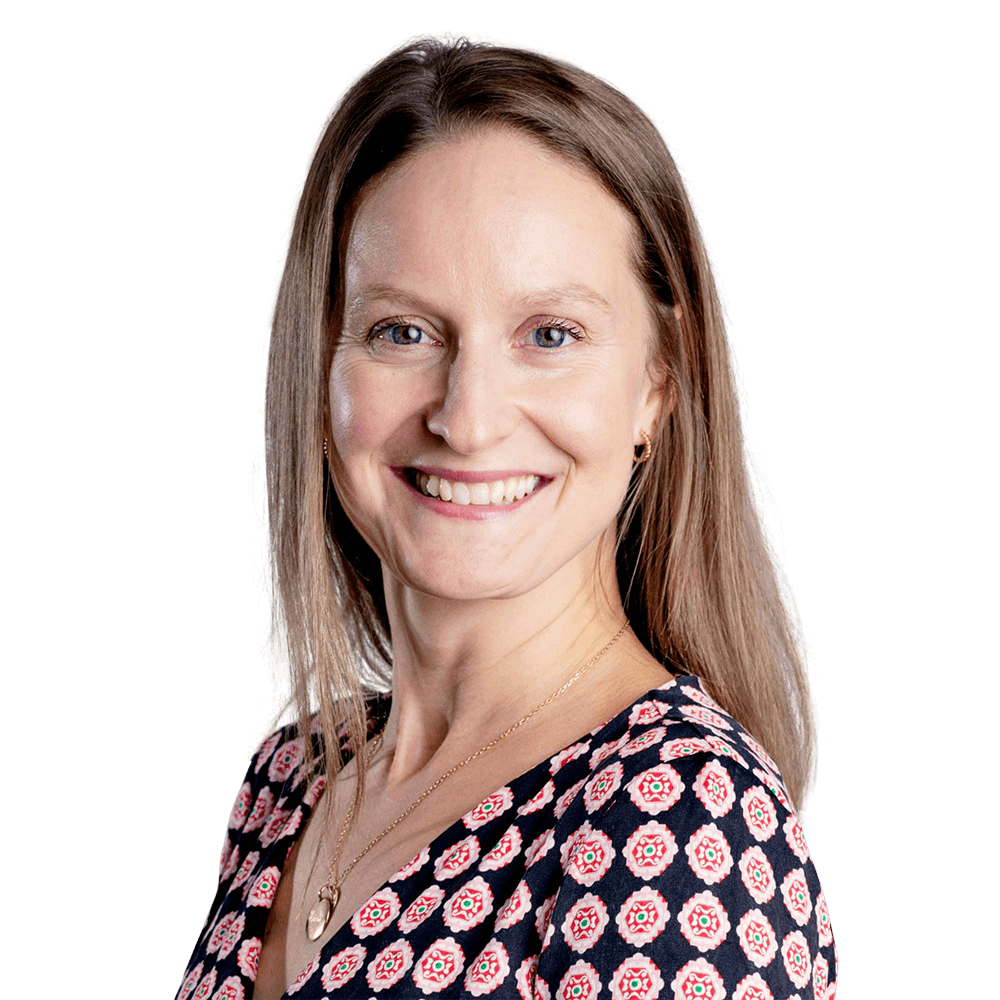
Anastasia Gorokhova
Partner, UK
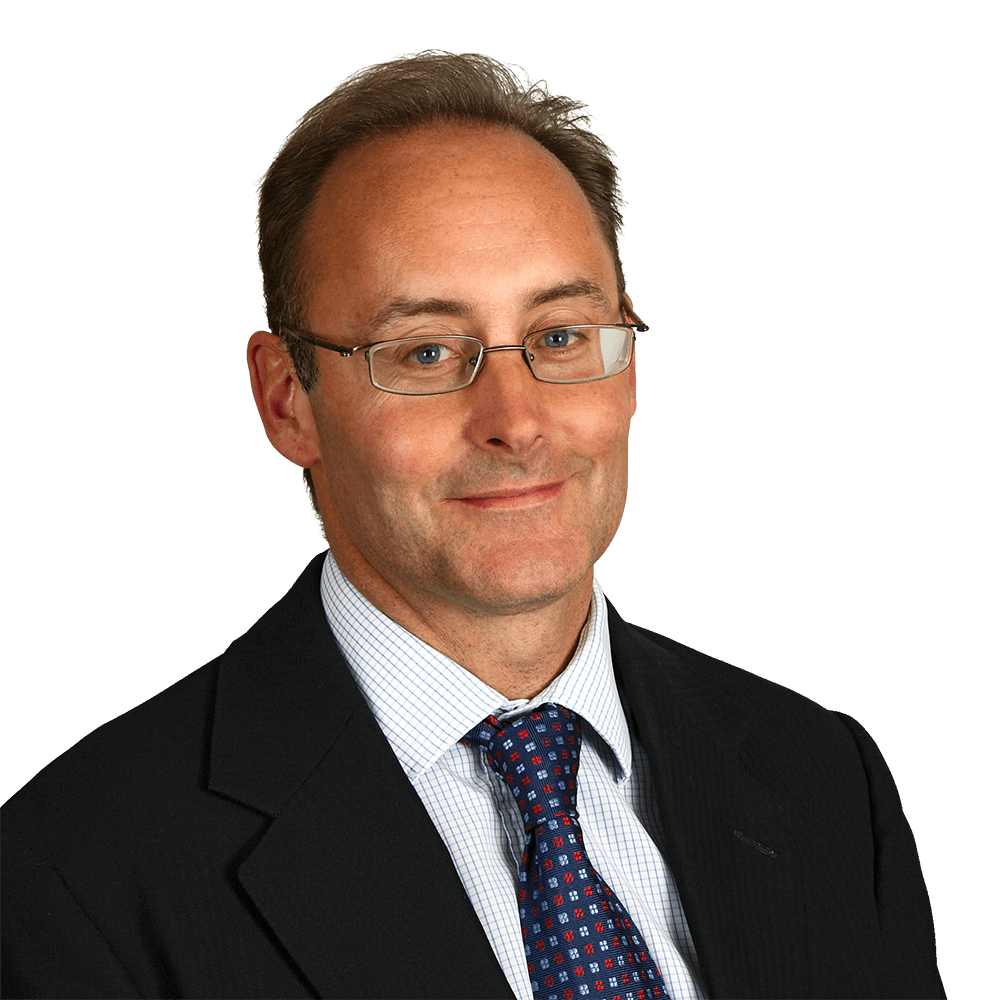
Paul Matthews
Partner, UK
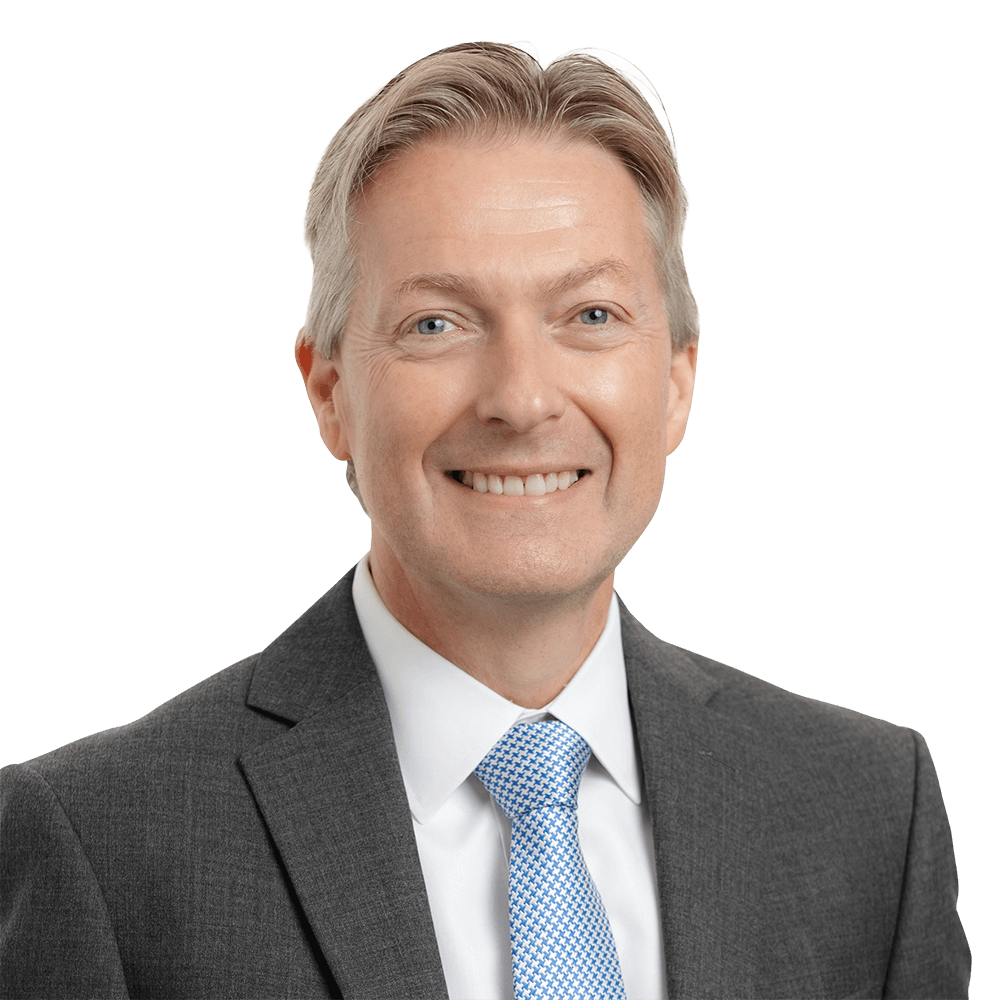
Steve Wilson
Managing Partner, US
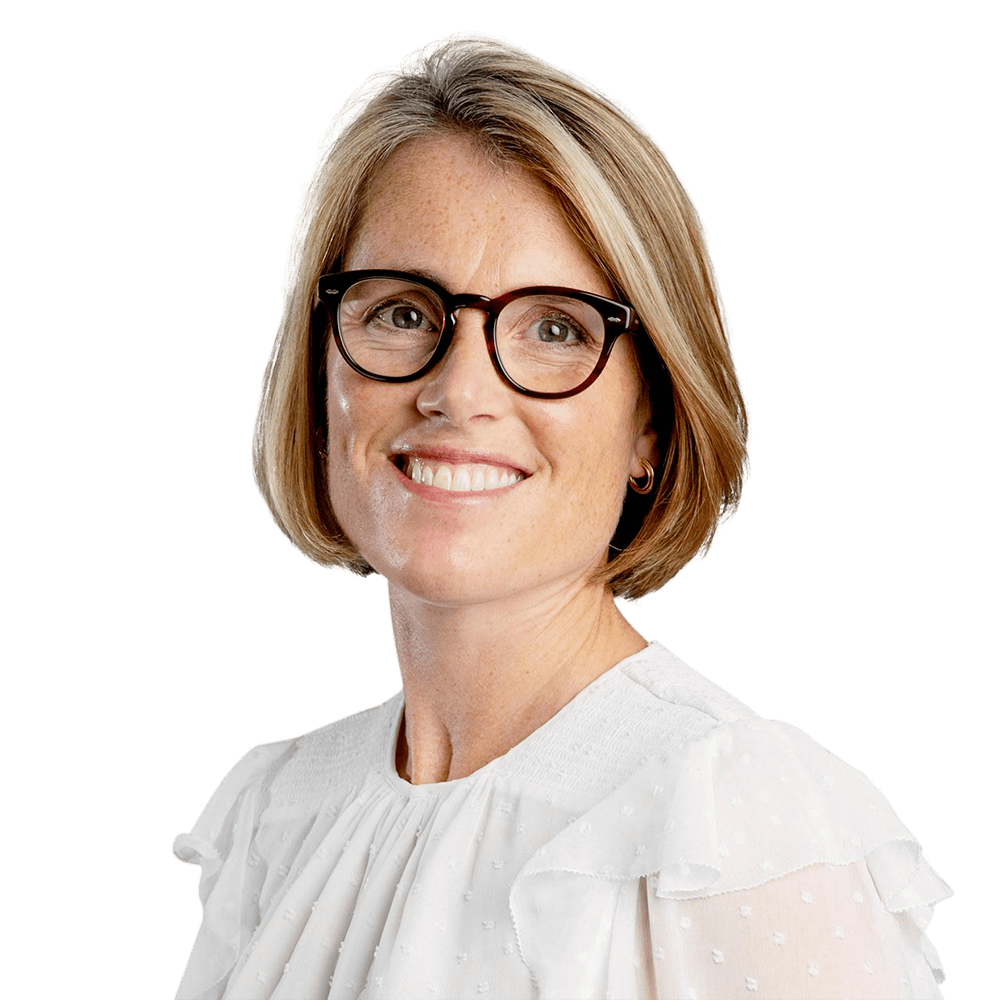
Alice Conway
Partner, UK
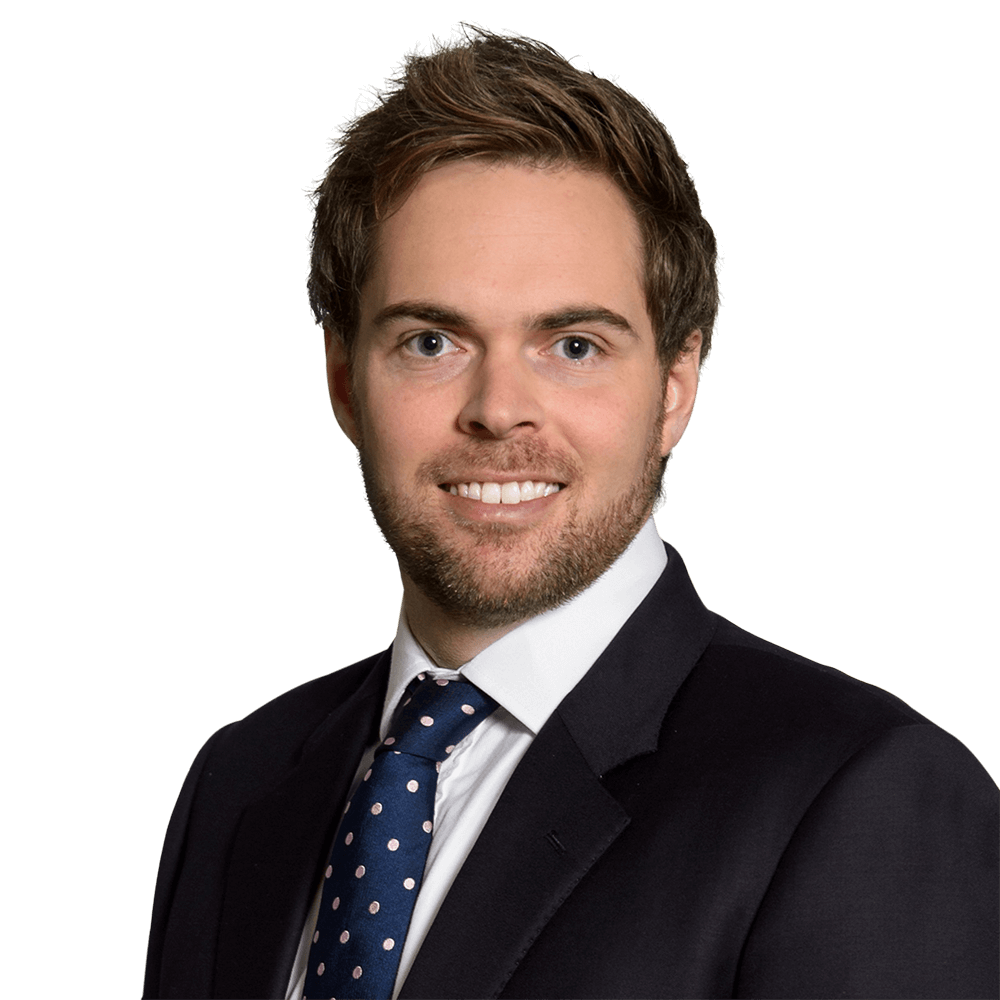
Chris Yeo
Partner, UK
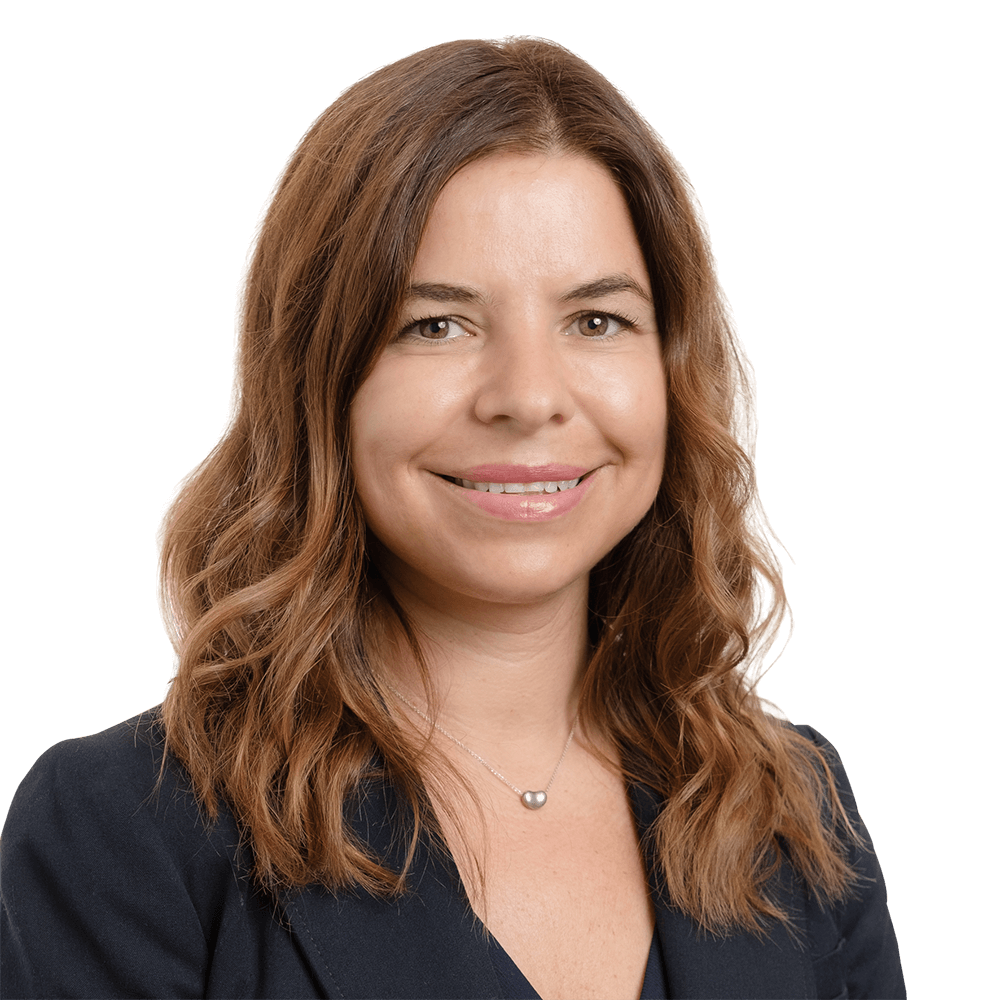
Kelly Harlick
Senior Vice President, International, US
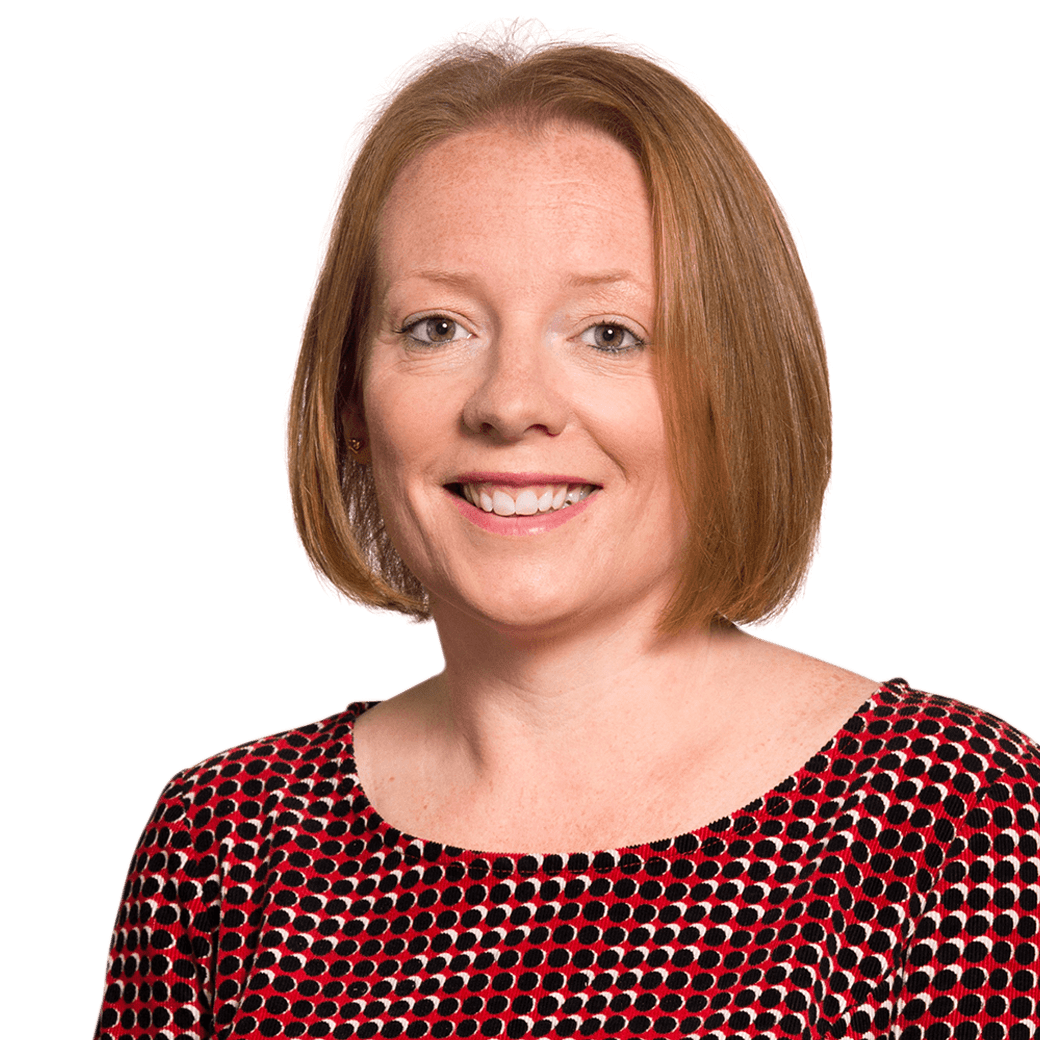
Michelle McGurl
Partner, Practice Group Head for Projects, Finance and Real Estate, UK
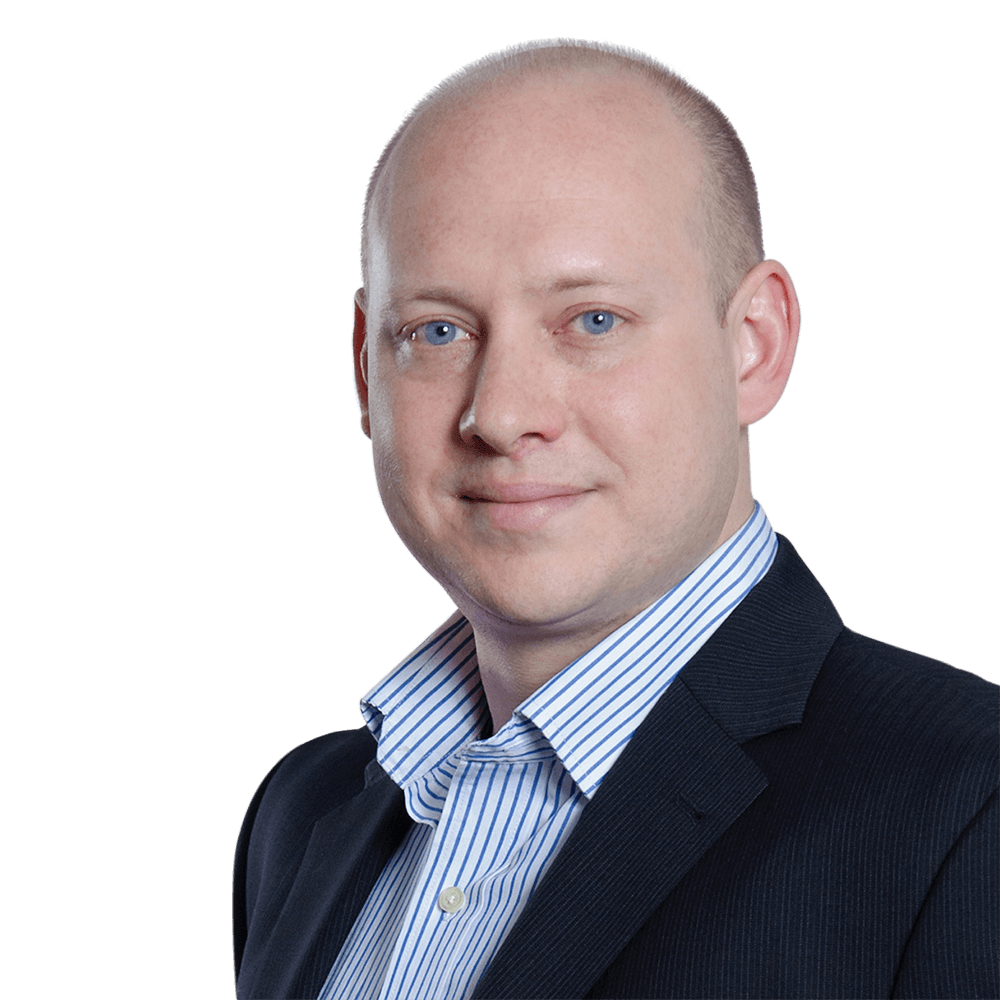
Simon Jones
Partner, UK
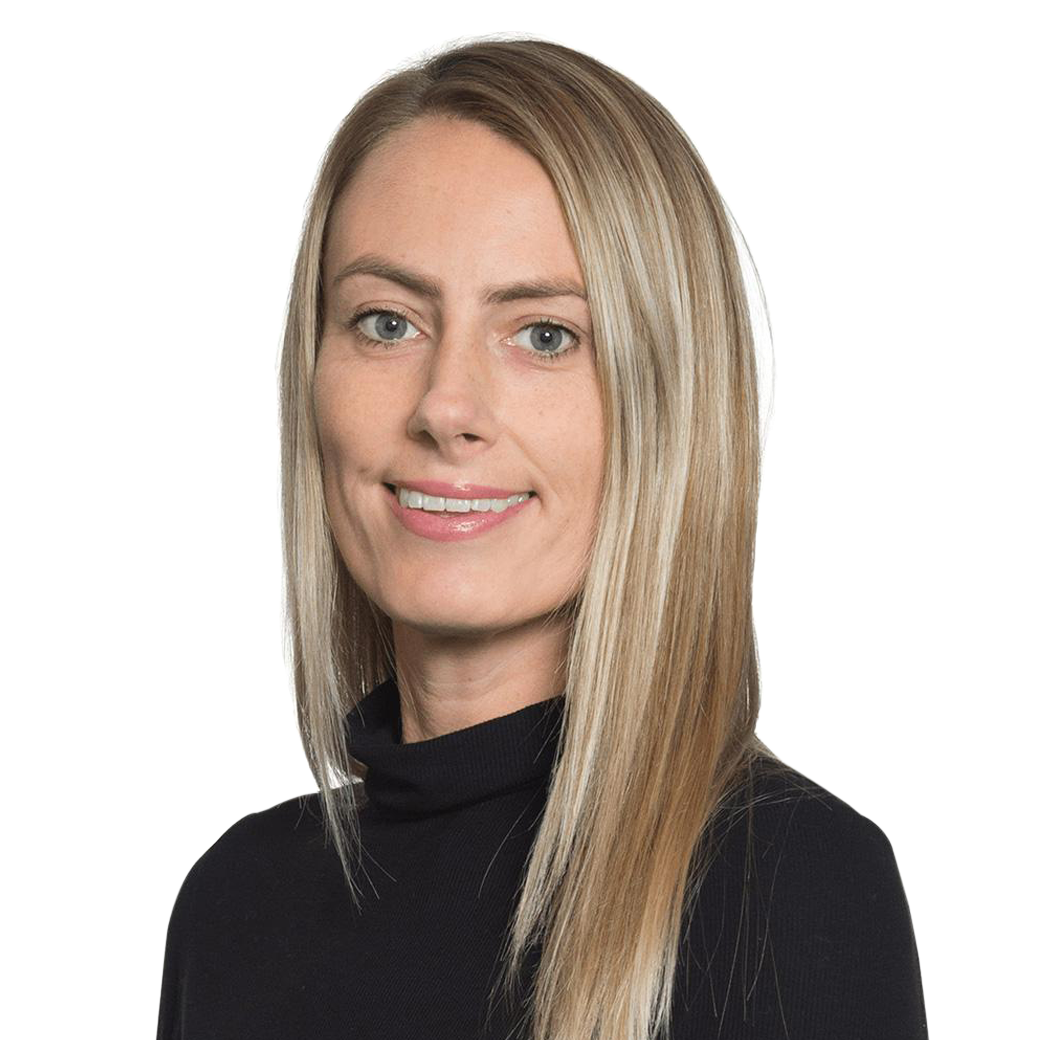
Sarah Moran
Senior Associate, UK
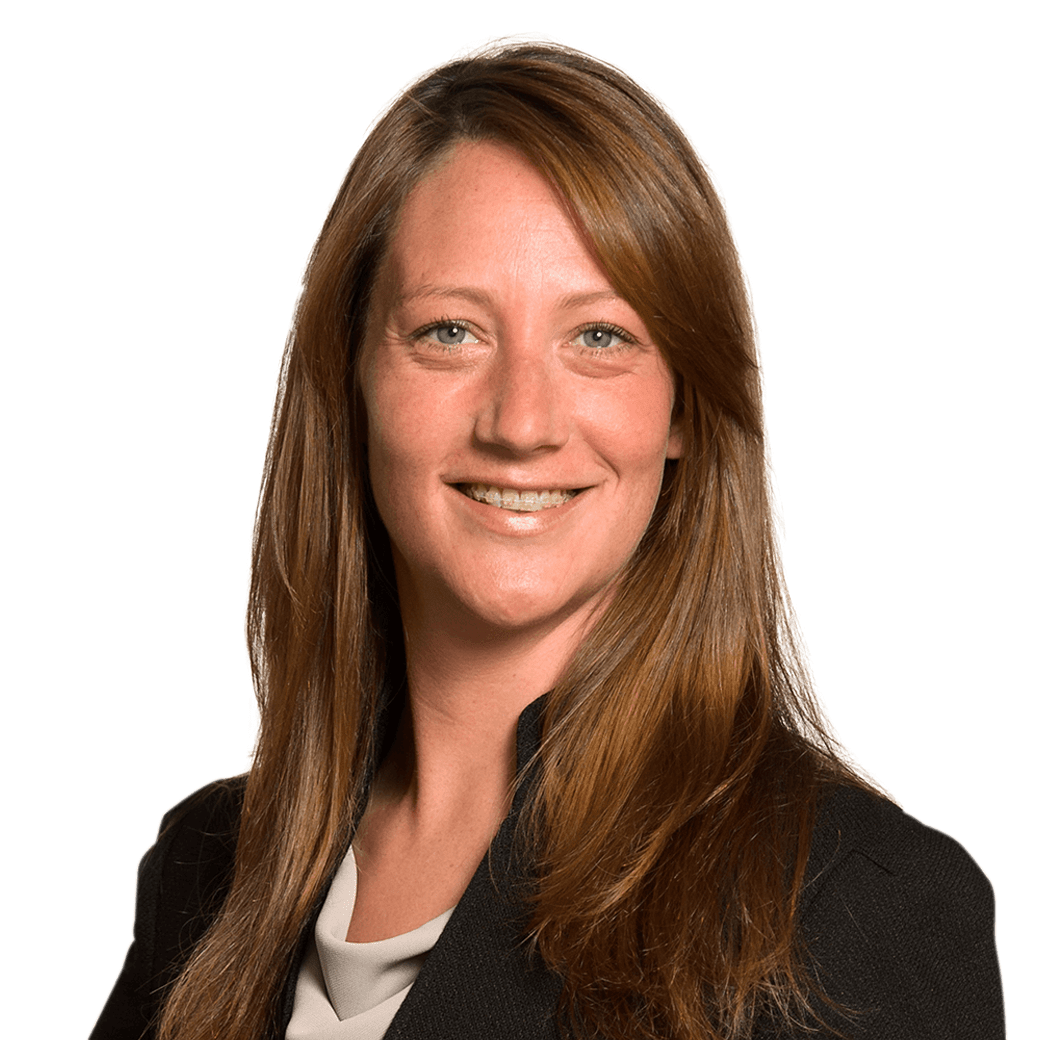
Georgia Lythgoe
Senior Associate, UK
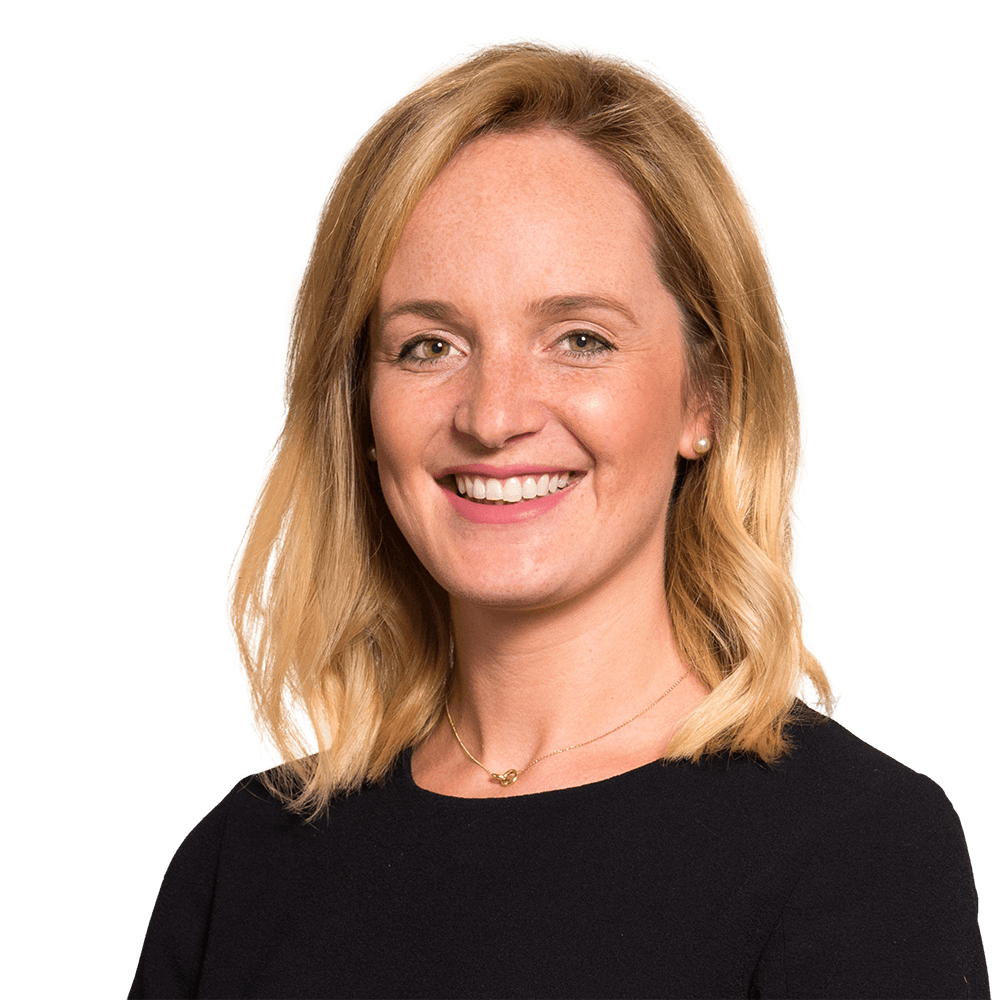
Julia Jolley
Associate Director, UK
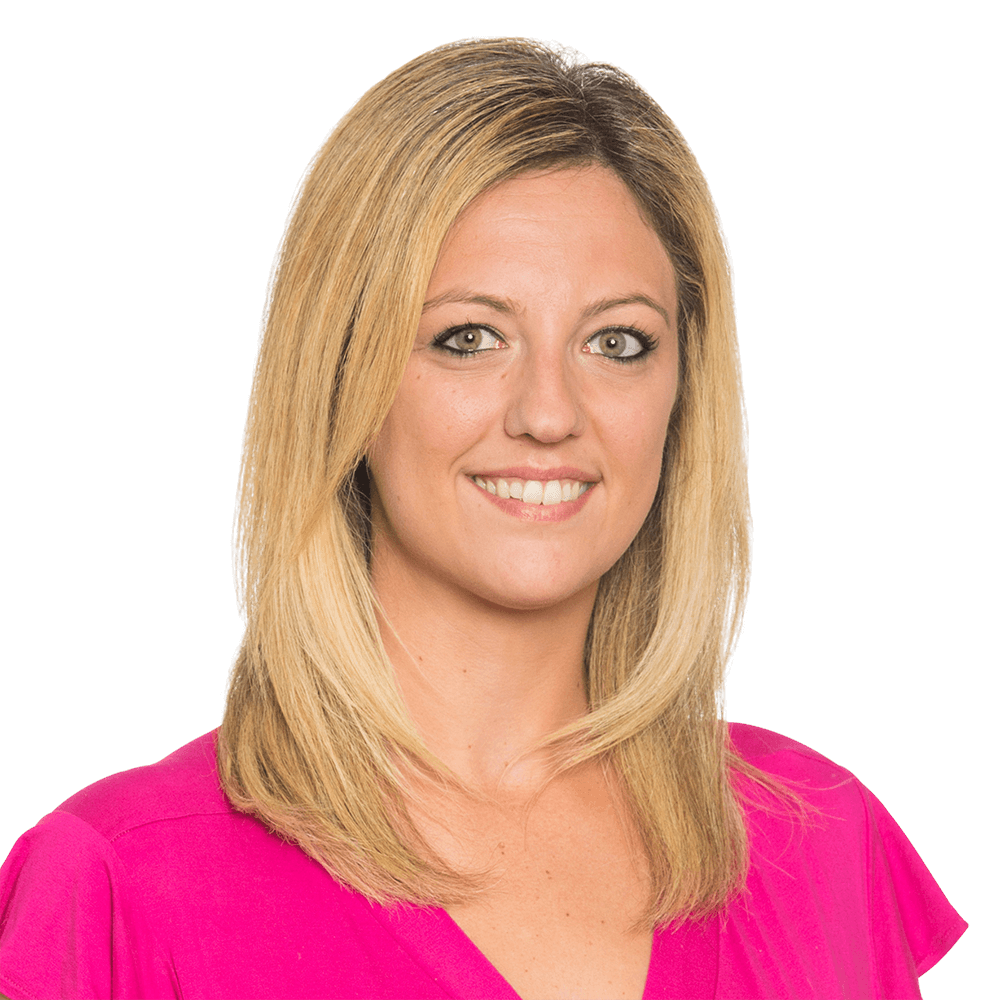
Joanna Risdale
Associate Director, UK
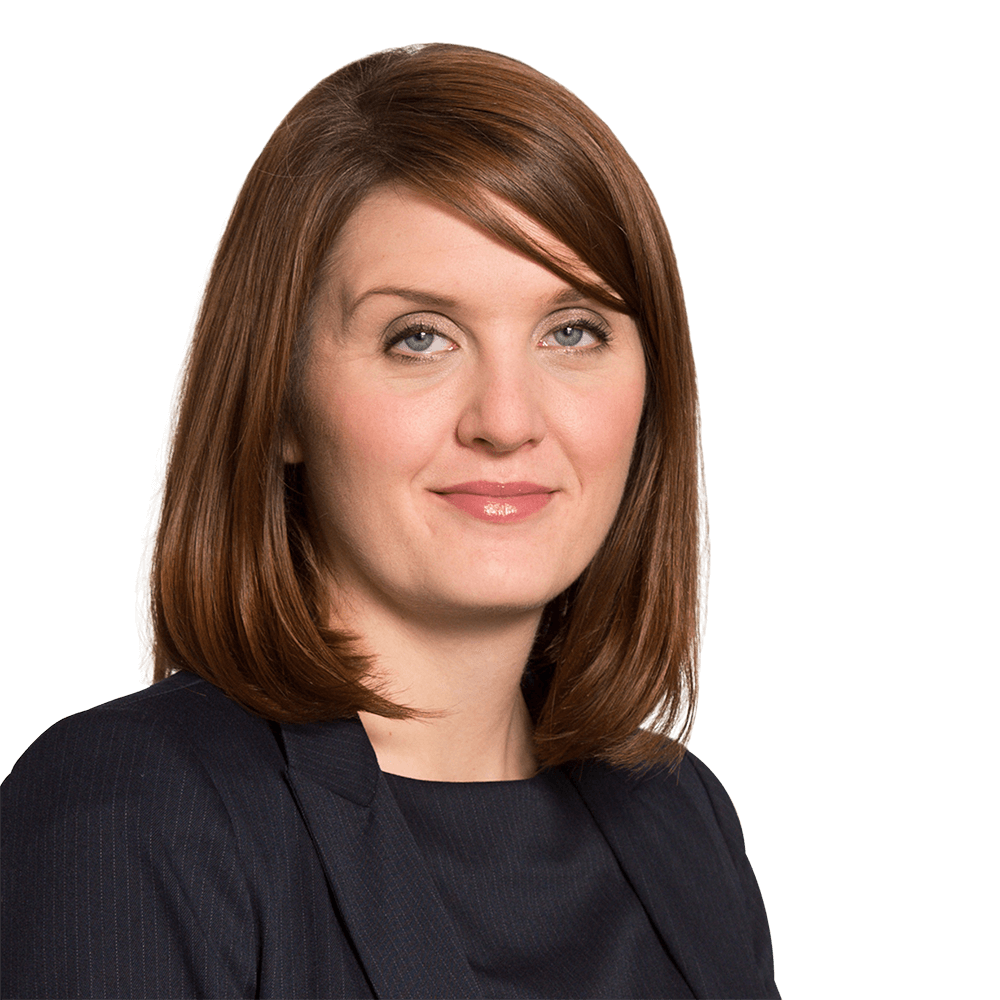
Sarah Beard
Legal Director, UK
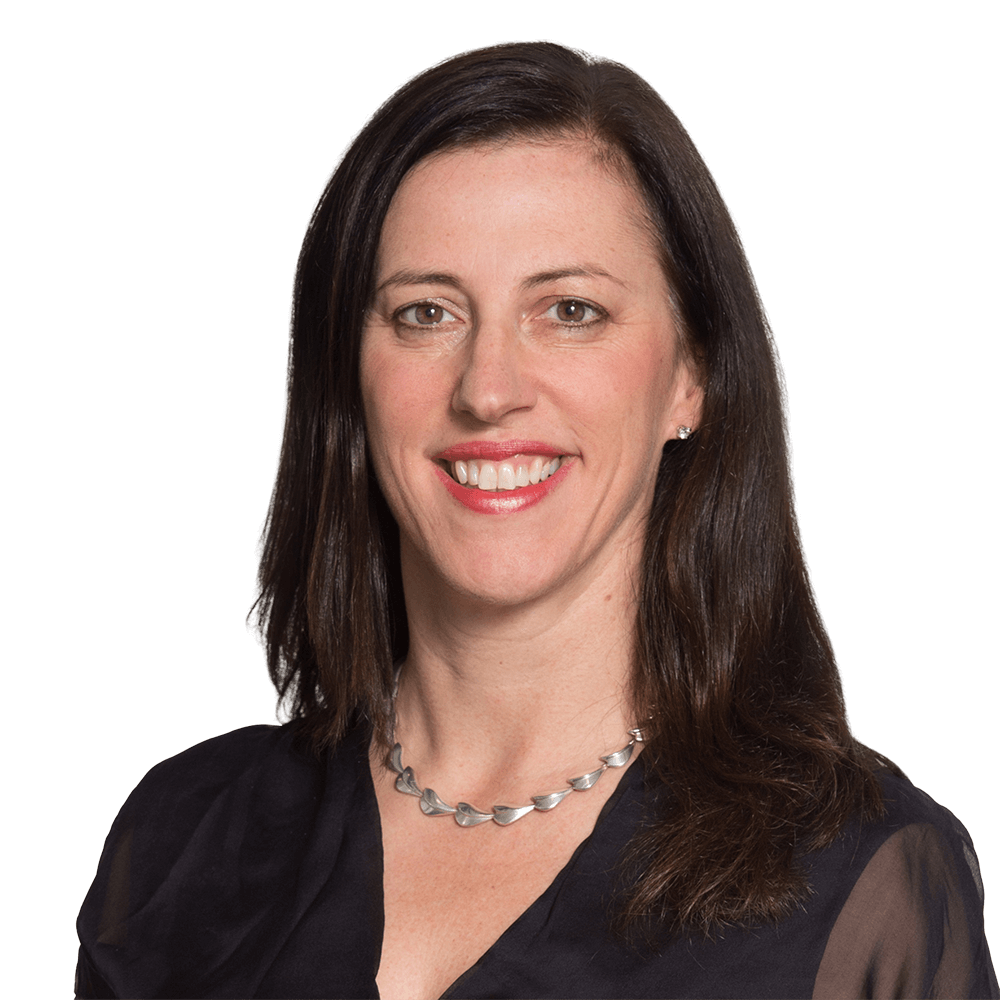
Jo Footitt
Partner, UK
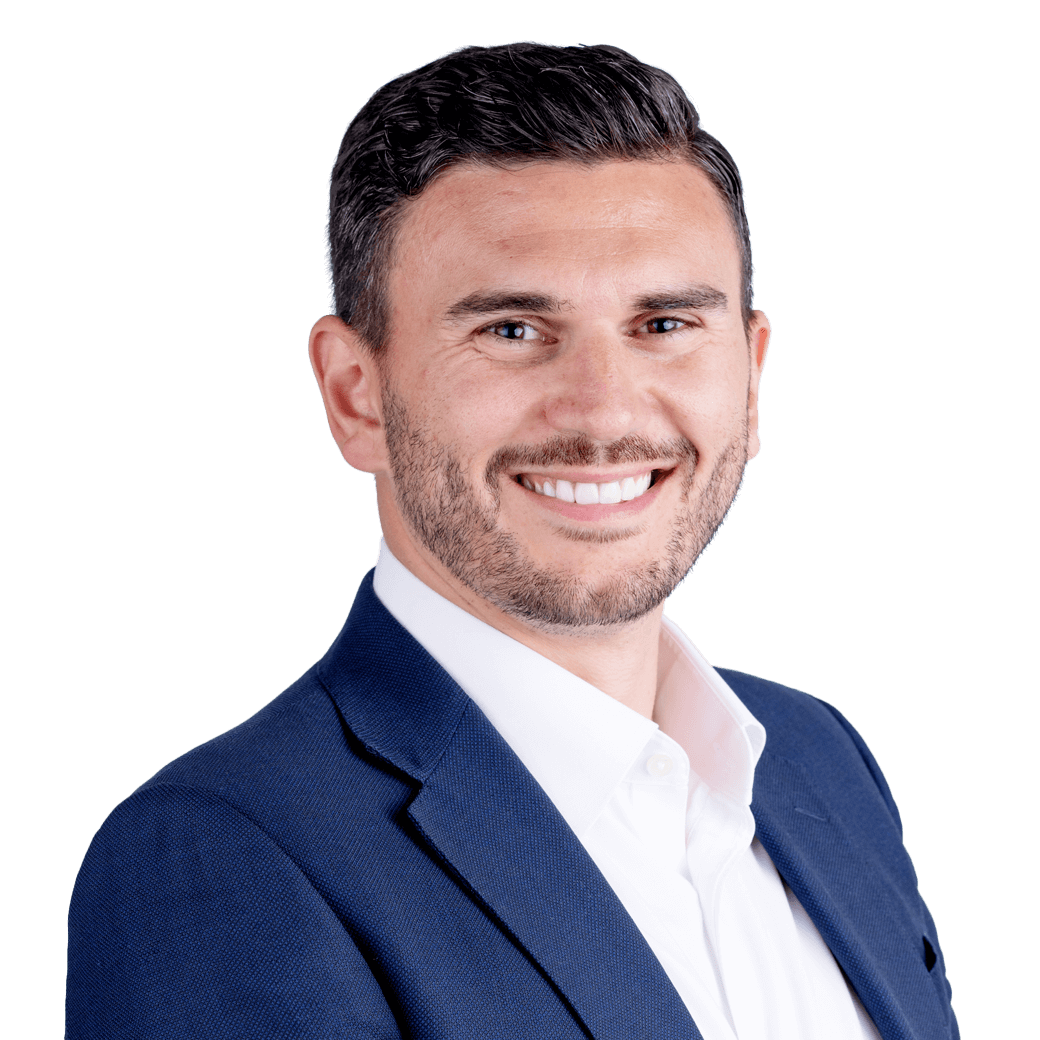
James Taylor
Partner, UK
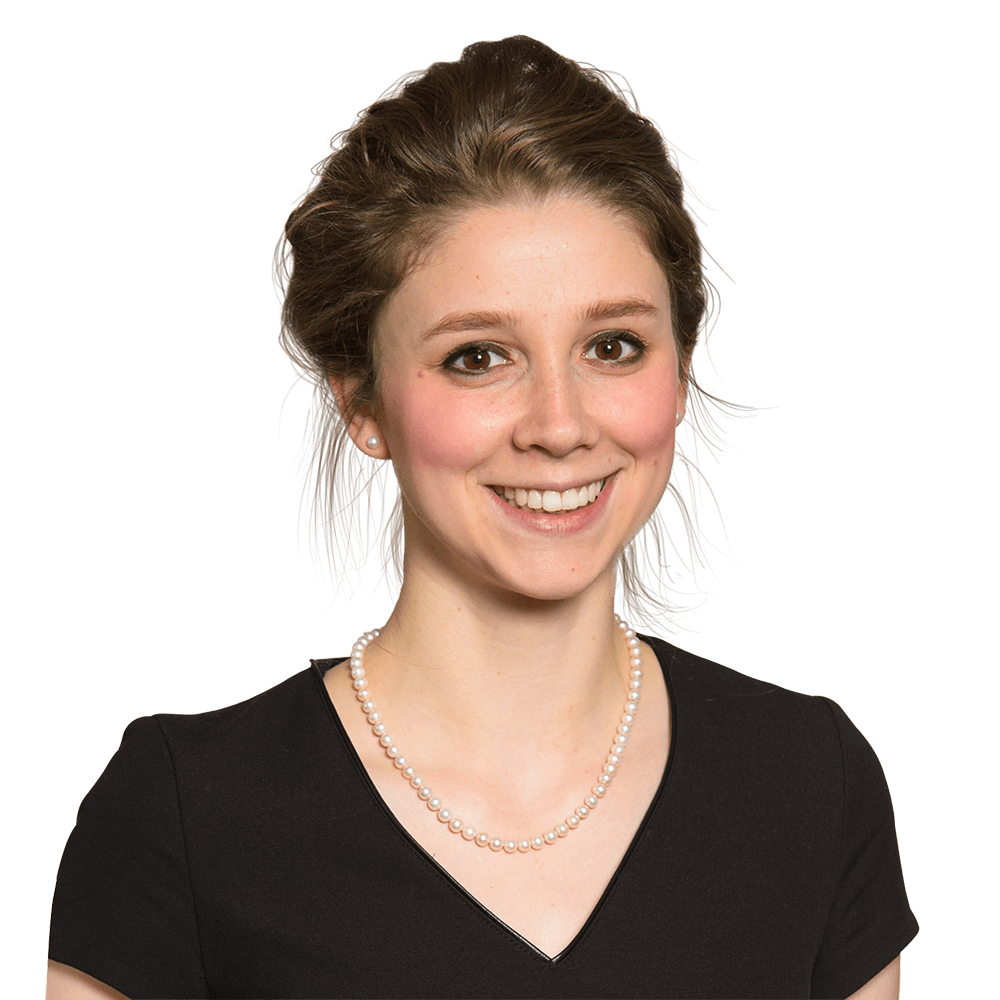
Rose Robson
Senior Associate, UK
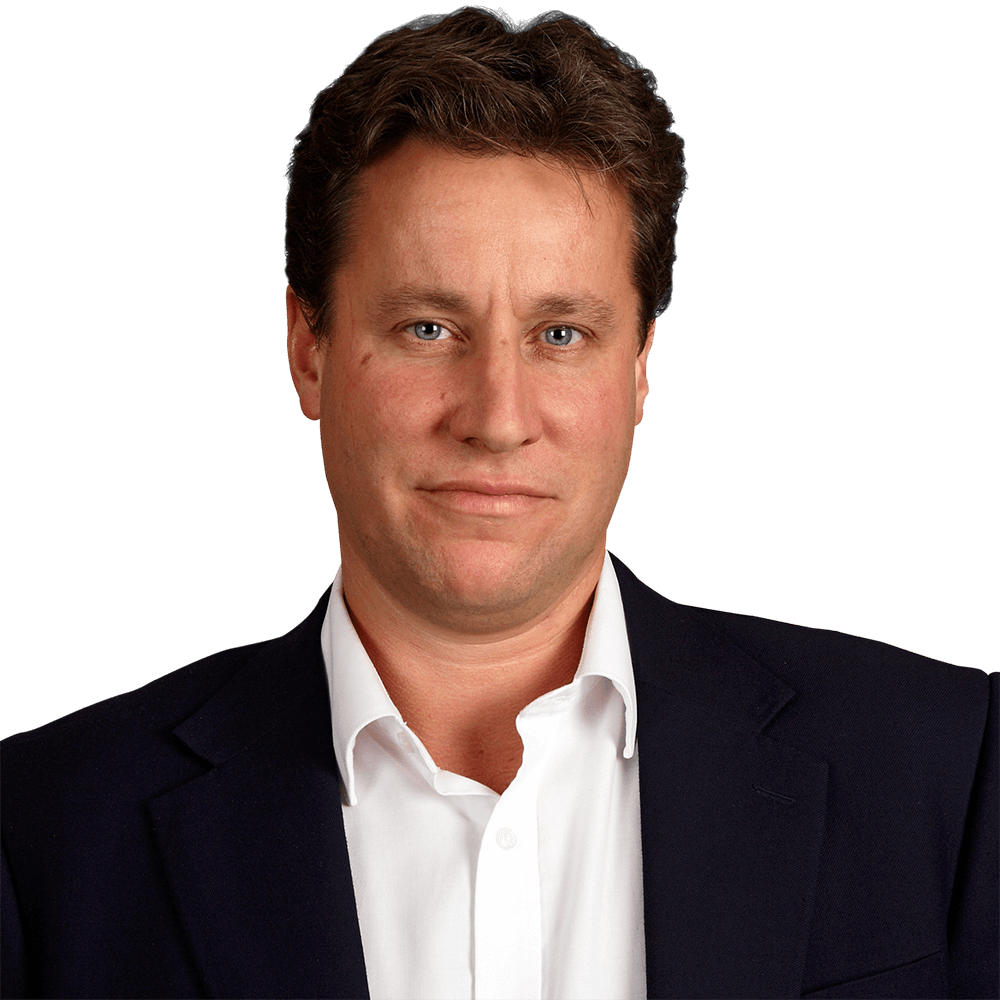
David North
Partner, Head of purpose built student accommodation, UK
How we've helped our clients

Limejump
Advised the shareholders of Limejump on the sale of the Company to Shell Energy.
Optimisation and dispatch services
Advising a leading energy tech company on its innovative optimisation and dispatch services contract suite for batteries and other distributed generators, including complex forward and within-day electricity trading arrangements.
Connected homes
Advising a multinational energy and services company on the rollout of their connected home offering. We are advising on commercial and regulatory law, including coordinating regulatory advice across multiple jurisdictions. From the outset, we realised that understanding their business drivers was key for this connected home offering and drew heavily upon our knowledge of how the digital consumer landscape varies across new territories. As such, we were able to provide prompt and comprehensive advice on all aspects of the project to support the client's objectives.
Advising a leading independent real estate consultancy
Advising a leading independent real estate consultancy in obtaining data from a major Singapore-based telecom and applying data analytics to help the Real Estate Investment Trust Managers of a multinational alternative asset management company optimise their retail mix and increase traffic.
Limejump
Advised the shareholders of Limejump on the sale of the Company to Shell Energy.
Optimisation and dispatch services
Advising a leading energy tech company on its innovative optimisation and dispatch services contract suite for batteries and other distributed generators, including complex forward and within-day electricity trading arrangements.
Connected homes
Advising a multinational energy and services company on the rollout of their connected home offering. We are advising on commercial and regulatory law, including coordinating regulatory advice across multiple jurisdictions. From the outset, we realised that understanding their business drivers was key for this connected home offering and drew heavily upon our knowledge of how the digital consumer landscape varies across new territories. As such, we were able to provide prompt and comprehensive advice on all aspects of the project to support the client's objectives.
Advising a leading independent real estate consultancy
Advising a leading independent real estate consultancy in obtaining data from a major Singapore-based telecom and applying data analytics to help the Real Estate Investment Trust Managers of a multinational alternative asset management company optimise their retail mix and increase traffic.
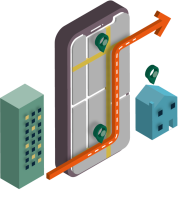
Explore Urban Dynamics
Real estate
The rollout of UK Electronic Communications Code changes continues one year on
What impact are new rules that have been introduced since last December having on network operators and site providers?
26/10/2023
Read time
3m
Real estate
Are modern methods of construction in the UK more sustainable?
Can modern methods of construction, such as off-site manufacturing, help to reduce carbon emissions generated in the construction of new...
27/04/2023
Read time
6m
Urban Mobility
Is the UK moving towards low carbon urban mobility solutions?
The UK government has indicated that it may legislate to ensure e-scooters sold in the UK meet certain safety standards...
22/11/2022
Read time
5m
Future of work
What does hybrid working mean for your company’s carbon footprint?
There is not yet a consensus about the best way for businesses to measure and address carbon emissions from remote...
08/11/2022
Read time
4m
Future of work
How businesses can benefit from nurturing a green workplace
Occupying greener premises can not only assist with business's sustainability targets but also help with the recruitment and retention of...
01/11/2022
Read time
4m
Urban Dynamics
Low carbon skills inhibiting smart city development: a chicken and egg situation?
To meet its carbon emissions targets, the UK needs to do more to decarbonise its cities – and this requires...
17/09/2022
Read time
3m
Urban Dynamics
Smart cities pose 'fit for purpose' questions for data regulation
The EU and UK need to balance the protection of individual rights and allowing for innovation in data-intense environments
17/08/2022
Read time
7m
Regulatory and compliance
EU nature restoration law set to boost biodiversity across Europe
New proposals to introduce legally binding targets for Member States on the restoration of habitats
05/07/2022
Read time
4m
The Built Environment
Is consent the hallmark of ethical data use in a real estate context?
As the world we live, work and play in becomes ever smarter and more digital, the property sector has a...
16/06/2022
Read time
1m
Data-driven business models
Challenging the environmental impact of data-driven business models
Net-zero goals have become a strategic priority for many businesses and so the carbon footprint of data-driven business models has...
14/06/2022
Read time
5m
Data-driven business models
Digital twins in the built environment
Digital twins can offer powerful insights into the built environment, including ensuring optimised energy use or enabling visualisations of environmental...
14/06/2022
Read time
12m
Sustainable disruption: 12 decarbonising technologies for cities
Decarbonising technologies for cities: Warsaw case study
New research | Commissioned by Osborne Clarke | Written by Economist Impact
15/03/2022
Read time
9m
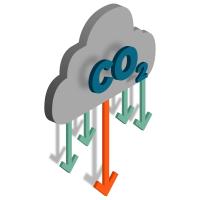
Decarbonisation
Climate change poses a significant challenge to our planet, our personal lives and our businesses.
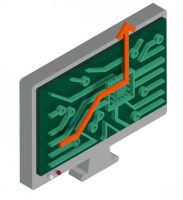
Digitalisation
Right now there’s probably at least one area of your business facing transformative change driven by technology or digital risk.
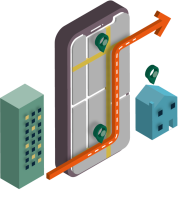
Urban Dynamics
We're here to help you negotiate the legal challenges you'll face as our cities change.